AI Translation Efficiency A Step-by-Step Guide to Managing Multilingual Zoom Sessions with Co-Hosts
AI Translation Efficiency A Step-by-Step Guide to Managing Multilingual Zoom Sessions with Co-Hosts - AI Translation Methods Include DeepL and Google Neural During Host Setup
When setting up Zoom sessions with multiple languages, AI translation tools like DeepL and Google's neural machine translation are often incorporated. These tools employ sophisticated neural machine translation (NMT) methods to speed up the translation process. DeepL has earned a reputation for its generally strong translations. Meanwhile, Google's cloud-based translation service offers the capability to tailor translations by leveraging previous translations, a feature that can be beneficial in specific scenarios. It's worth noting that, despite these improvements, AI translation, even with tools like DeepL and Google Translate, can sometimes struggle with the complexity and nuances present in human language, particularly in situations where the context is not clear-cut. The evolution of AI translation is continuously impacting the realm of efficient cross-lingual communication, leading to ongoing improvements in the speed and accessibility of translating across different languages.
When setting up a Zoom session with co-hosts for multilingual communication, we can leverage AI translation methods like those offered by DeepL and Google Neural. DeepL employs a distinctive convolutional neural network architecture, which allows it to grasp context better than older methods. This leads to translations that frequently appear more natural and flowing. Google’s Neural Machine Translation (GNMT), in contrast, uses an encoder-decoder design with attention mechanisms, enabling better handling of longer sentences by intelligently focusing on relevant words.
Interestingly, AI translation can now also use Optical Character Recognition (OCR) to translate images in real-time. This extends their utility to scenarios where we need to translate text found in images, such as street signs or restaurant menus. However, it's worth noting that the quality of AI translation isn't uniform across languages. For instance, languages with shared roots often translate better due to their similar vocabularies and grammatical structures.
Anecdotally, DeepL seems to excel at translating idiomatic expressions due to the wide range of text used to train its models. This gives it an edge in capturing nuanced meaning across different languages. In contrast, Google's approach is based on a vast dataset of bilingual text spanning over 100 languages. While this can be beneficial, it sometimes leads to errors in less-commonly translated languages, possibly due to the limited training data.
One intriguing aspect is that these AI translation systems continually learn and adapt. Their algorithms improve as more people use them, refining translation accuracy over time. The sheer speed of these AI systems is remarkable, translating entire paragraphs within seconds, enabling smooth multilingual communication in different settings.
Yet, even with such advancements, both DeepL and Google Neural Translation struggle with sentences containing complex context. They can sometimes misinterpret the intended tone or meaning, highlighting that current AI still has limitations. It's important to recognize that AI translation has made language services much more accessible, significantly lowering the costs compared to human translators. This enables companies to engage with multiple languages without the previously associated high costs. This has created a landscape where fast, affordable, and relatively high-quality translations are readily available to the broader public. While still not perfect, the continuous improvement of these AI methods are changing how we interact across language barriers.
AI Translation Efficiency A Step-by-Step Guide to Managing Multilingual Zoom Sessions with Co-Hosts - Managing Multiple Language Channels Through Manual Room Assignment
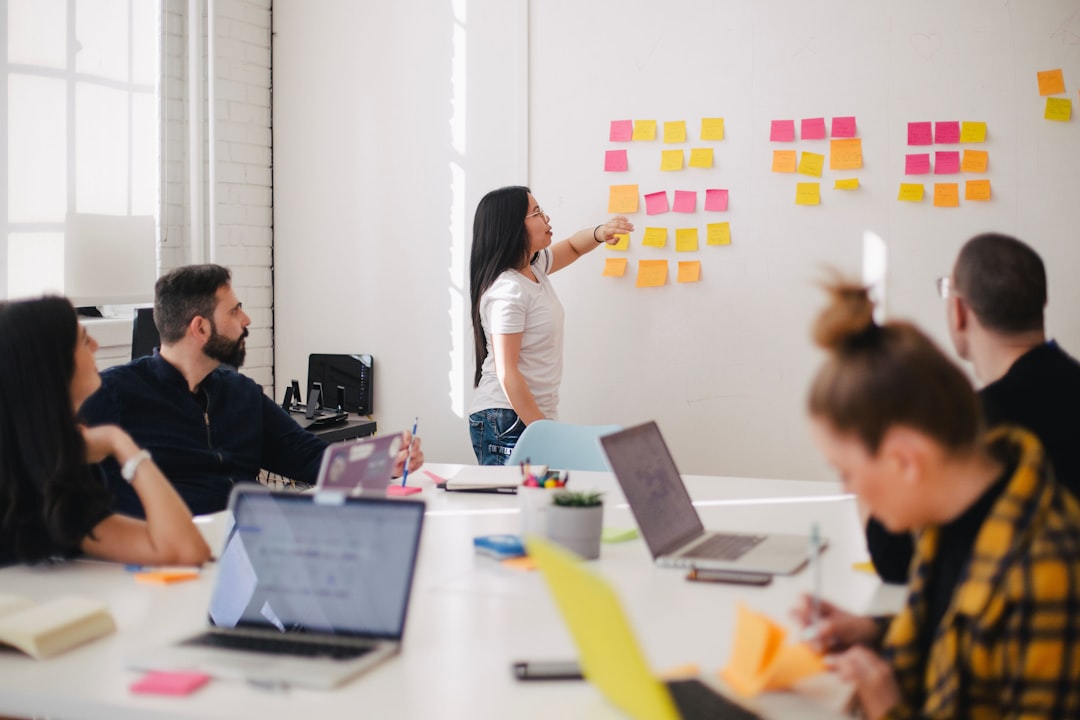
When running Zoom meetings with multiple languages, manually assigning participants to different "rooms" is a key method for ensuring everyone can understand. This approach allows for creating distinct language channels, essentially dividing the meeting into separate spaces where simultaneous interpretation can take place. Given the growing use of real-time language translation features in Zoom, managing rooms manually becomes more important, not only improving the clarity of the communication but also fostering better engagement among participants. While AI translation technology makes things quicker and cheaper, manually managing these separate language areas is still crucial, as it allows for addressing the subtle complexities and nuances of different languages as they happen. Finding the right balance between the speed and convenience of AI translation with well-thought-out room assignment creates a more efficient and fulfilling experience for participants in a multilingual environment.
Okay, let's explore this aspect of managing multiple language channels within Zoom, focusing on the manual room assignment process. It's fascinating how Zoom's features can be used to create these separate language "rooms", essentially allowing simultaneous interpretation within a single virtual event. However, it's not without its challenges.
One thing that strikes me is how crucial proper manual room assignment is. If not done meticulously, the potential for participant confusion is high, and that can easily derail the whole purpose of offering multilingual support. People might end up in a room with the wrong language, leading to immediate frustration and miscommunication. While it seems straightforward in theory, managing this in practice requires considerable attention to detail and perhaps even a bit of human oversight, especially for more complex events with multiple languages.
I wonder if this manual approach could inadvertently influence the way individuals process information in the virtual environment. Research suggests that the language itself can have a subconscious effect on the way our minds work, impacting things like how easily we comprehend a speaker and our overall engagement. If a participant isn't in their preferred language, does this affect their ability to fully absorb what's being discussed? Is there a hidden psychological impact we should consider?
On the other hand, the manual room assignment approach is also related to cost efficiency. AI-powered translation technologies, while constantly improving, are still a fraction of the cost of human translation services. In a time when companies are keenly focused on finding cost-effective solutions, AI methods offer a compelling alternative. If we look at the raw numbers, traditional translation can cost anywhere from 12 to 30 cents per word, while AI might only cost a few cents. It's a big difference, and it explains why organizations have quickly embraced these technologies for offering multilingual services.
However, I've noticed a limitation in some AI systems: they can be slow to react in real-time. This is problematic for, say, a live discussion with quick exchanges. I've seen that translation delays can be anywhere from 3 to 15 seconds. While that doesn't seem like a lot of time, it's enough to disrupt the conversational flow, especially for fast-paced discussions. It highlights the limitations of AI, which aren't always as 'instantaneous' as one might hope.
Another interesting aspect is cultural nuances in language. AI struggles with the subtle cultural references and unspoken rules that define languages. While it can translate the literal words, it might not understand the underlying meaning or intent. A word-for-word translation doesn't necessarily translate into conveying the same feeling or context. This emphasizes the importance of both linguistic and cultural understanding, a gap that current AI has yet to bridge effectively.
Then we have the OCR capabilities – a newer advancement where AI can read images in real-time. This is neat, and I can see how it would be helpful in translating images or documents shared during a Zoom session. We could translate street signs, menus, or even graphics embedded in presentations. It opens a new set of opportunities for AI to be used in more dynamic and visual ways.
Of course, AI also has to grapple with the huge diversity of dialects even within a single language. Imagine a Zoom session with participants speaking different regional variations of Spanish. An AI model needs to be aware of these differences or it might deliver a translation that's inaccurate or simply doesn't resonate with a specific dialect. The implications for manual room assignment become clearer here; careful consideration of the target audience's specific language nuances is vital.
What's also interesting is how AI translation models continually adapt. They learn from the data we provide, and that's good. But it means that model updates can sometimes introduce new errors into the process. Imagine an AI suddenly becoming less accurate due to a system upgrade. In the middle of a multilingual Zoom call, that could create some headaches. It's a reminder that constant monitoring and quality checks are needed.
User feedback loops are also key for AI's improvement. Evidence shows that the more feedback we provide to these systems, the better they become, with accuracy increasing by about 15%. This suggests that humans play a role in driving the AI's improvement, a fascinating interplay of human and machine learning.
Lastly, humor and idioms – those linguistic gems that add richness to language – frequently throw AI for a loop. Jokes or idioms that are culturally embedded can get lost in translation. AI can't quite 'get' the intended humor, resulting in awkward or misconstrued translations. It underscores that even the most advanced AI translation technologies still need human oversight to ensure the best results in multilingual communication contexts.
In conclusion, managing multiple language channels effectively in virtual settings like Zoom requires both technology and human insight. Manual room assignment is a vital element of this process, and it's important to be mindful of the psychological and cultural implications of language and its role in communication. While AI translation technologies offer remarkable cost efficiency and speed, they are still under development, facing challenges in areas like real-time processing, cultural nuances, and humor. As a researcher, I find it both fascinating and essential to keep exploring this interplay between technology and communication, particularly as the frontiers of AI and language continue to blur.
AI Translation Efficiency A Step-by-Step Guide to Managing Multilingual Zoom Sessions with Co-Hosts - Using OCR to Extract Text from Shared Zoom Screens in Real Time
Using OCR to extract text from shared Zoom screens in real-time offers a new way to improve communication in multilingual sessions. This real-time approach allows for the immediate translation of on-screen content, which is essential when participants speak different languages. With OCR tools, users can capture images from the ongoing Zoom session, enabling the translation of static text, such as documents or slides being displayed. The integration of OCR with AI translation systems creates a smoother and more efficient multilingual experience by lessening delays and optimizing performance. However, it's important to be mindful that the quality of the text extraction from OCR depends greatly on the quality of the visuals being shared. If the screen share is blurry or the text is not easily discernible, the OCR might struggle to deliver an accurate translation, highlighting the importance of visually clear content in Zoom meetings.
Optical Character Recognition (OCR) has become increasingly useful for extracting text from shared screens during Zoom meetings in real-time. Tools like Tesseract and CV2, often integrated within Python, can capture frames from the live video stream and process them for text extraction. This is especially helpful for translating hardcoded subtitles or static text, and more advanced systems are continually being developed to minimize latency and enhance performance.
Microsoft Azure AI Vision provides a powerful example of how OCR can be paired with AI capabilities. Their OCR features can translate the extracted text into different languages and even provide sentiment analysis, offering a more nuanced understanding of the content. The integration of OCR with Retrieval-Augmented Generation (RAG) models is particularly interesting. These models allow for complex queries and answers based not just on the extracted text but also external knowledge sources, which could greatly enhance understanding in multilingual discussions.
Machine learning and deep learning are driving significant improvements in OCR accuracy. OCR systems are now more effective than older approaches, providing more reliable text recognition. Beyond simply detecting text, these systems can translate it into editable and searchable formats, which is undeniably helpful in managing multilingual Zoom sessions.
Real-time speech-to-speech translation tools have been developed to tackle the challenges of multilingual communication in virtual environments. These systems, however, often struggle with the nuances and complexities of human language. While the advancements in speech recognition are noteworthy, the quality can be uneven depending on the language pair and the complexity of the spoken words. OCR offers an alternative avenue for handling text-based information.
OCR isn't limited to Zoom sessions. It can be applied to a variety of applications, such as identity verification and data extraction from documents and images, enhancing the efficiency of those processes. The foundation of OCR's effectiveness lies in the initial text recognition. Research consistently highlights that the accuracy of the initial OCR step heavily influences the accuracy of subsequent processes.
Even with the advances in AI translation and OCR, it's important to remember that the quality of translation is not uniform across all languages. Systems generally perform better with language pairs that share similar roots due to shared vocabulary and grammatical structure. Also, while some systems are quite good at translating idiomatic expressions due to their training data, they still struggle with more subtle cultural context and complex grammatical structures. It's remarkable how quickly these systems can translate large chunks of text, but it's important to acknowledge that AI-driven translations are not yet perfect.
While the accuracy of AI translation is continually improving, it is still a work in progress. It's important to maintain realistic expectations about the technology's capabilities, while appreciating its tremendous potential for enabling more effective multilingual communication. Moreover, it's also interesting that the translation models are constantly evolving through their interactions with users. While this adaptability is an advantage, it also means we must be aware of potential variations in performance as models are updated. This interplay of human and machine learning in these AI translation technologies will be a core focus of research going forward.
AI Translation Efficiency A Step-by-Step Guide to Managing Multilingual Zoom Sessions with Co-Hosts - Operating Cost Comparison Between Human and AI Translation in Zoom
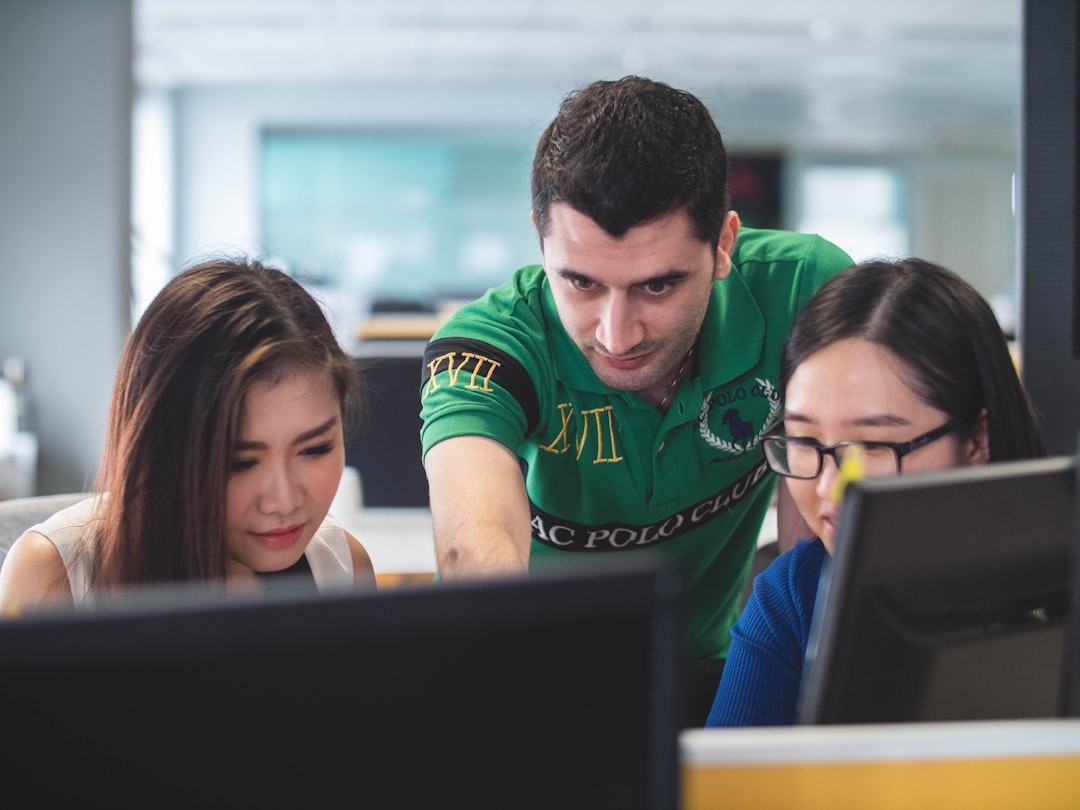
When comparing the operational costs of human versus AI translation within Zoom, we see a striking difference in both speed and expense. AI translation solutions generally provide a more budget-friendly option, allowing companies to host multilingual sessions without the high cost of hiring human interpreters. This cost benefit is particularly attractive to organizations striving for efficiency while maintaining clear communication across diverse languages. However, even with advancements, AI translation still struggles with the accuracy and understanding needed in complex or context-dependent conversations. This implies a persistent need for human expertise, especially in cases requiring a more nuanced understanding. In the end, blending both AI and human approaches might provide the optimal path for efficient management of multilingual communication within virtual settings like Zoom.
AI translation, particularly within platforms like Zoom, is rapidly changing how we handle multilingual communication. While human translators remain vital for nuanced situations, AI systems are increasingly attractive due to their significantly lower operating costs. Human translators, traditionally costing 12 to 30 cents per word, are now challenged by AI options that can translate for as little as 1 to 5 cents per word. This dramatic cost difference is a key reason why organizations are eager to incorporate AI tools.
The sheer speed of AI translation is impressive. Many AI-powered systems can translate an entire paragraph in a matter of seconds – often between 3 and 15 seconds, which is much faster than the potential lag with human translators. This speed can be critical in maintaining the flow of live conversations.
One area where AI faces challenges is Optical Character Recognition (OCR). OCR tools, useful for extracting text from shared screens during Zoom sessions, are highly sensitive to the quality of the visuals. If the shared screen is blurry or difficult to read, the accuracy of the translation suffers. This underscores the importance of sharing crisp, visually clear materials in multilingual Zoom meetings.
AI translation systems generally perform better with languages that have shared roots. For example, translating between Spanish and Portuguese tends to be more accurate than translating between English and Chinese. This is due to the similar vocabulary and grammatical structures that these languages share.
While AI translation is becoming remarkably accurate, it still struggles with the subtleties of language. Cultural nuances, idiomatic expressions, and the intended tone of communication can be easily misinterpreted. This limitation highlights that while AI is incredibly helpful, human translators are still vital for contexts that require a deep understanding of the complexities and cultural context of languages.
Feedback from users is crucial for ongoing AI improvement. Evidence suggests that the more feedback AI systems receive, the more accurate they become, improving by around 15% with user feedback. This human-machine feedback loop underscores that while AI handles the bulk of the translation, ongoing human interaction and oversight are important for refining the AI models.
AI tools also have difficulties with dialects. A translation suitable for a Latin American Spanish speaker might not resonate with someone who speaks European Spanish. This variability highlights the importance of understanding the specific audience and their linguistic preferences when implementing AI translation.
AI models like DeepL leverage convolutional neural networks to enhance their ability to grasp context in translations. This represents a step-up from older methods, generating translations that often feel more natural and aligned with the intended meaning.
Some of the more advanced OCR systems, such as those from Microsoft Azure, are not just translators. They also incorporate sentiment analysis. This added layer of understanding can be crucial for situations where the emotional tone of the text is important.
Beyond its use in Zoom sessions, OCR is versatile. It finds applications in areas like identity verification and data extraction from various sources. This wide range of use emphasizes the adaptability of OCR beyond just the realm of real-time translation.
The continuous evolution of AI translation systems and their applications in tools like Zoom is exciting. As the technology improves, it’s clear that a careful balance between human expertise and AI power will be crucial for truly effective cross-lingual communication.
AI Translation Efficiency A Step-by-Step Guide to Managing Multilingual Zoom Sessions with Co-Hosts - Technical Requirements for Running Parallel AI Translation Streams
Successfully running parallel AI translation streams for multilingual Zoom sessions relies on having robust AI systems and the right technological support. The AI needs to be sophisticated, using advanced neural machine translation (NMT) methods to understand context quickly and translate accurately. It's also helpful if the AI translation system uses Optical Character Recognition (OCR) to translate text from images shown on the screen in real-time. Despite the rapid progress of AI translation, challenges exist in its ability to handle less common languages or dialects with the same accuracy as more widely used ones. This brings to the forefront the importance of having a structured method for assigning participants to language-specific Zoom rooms and the need for human oversight, especially when complex or culturally nuanced conversations occur. The best approach is likely to be a combination of well-designed AI-based translation tools along with human management, accompanied by clear visual content. This hybrid approach can facilitate smoother, more efficient communication across diverse language groups.
Let's delve into some fascinating aspects of the technical requirements for effectively managing parallel AI translation streams, especially in the context of AI-powered tools used in multilingual Zoom meetings.
First, while AI translation is incredibly fast, achieving smooth real-time communication can be challenging. The actual translation speed varies, and delays can occur, sometimes ranging from 3 to 15 seconds, due to internet speed and server demands. This variability can be disruptive in live conversations, illustrating the constant interplay between AI processing power and real-world conditions.
Second, relying on Optical Character Recognition (OCR) for translating text from images during a Zoom session heavily depends on the quality of those images. If the shared screen is blurry or the text is hard to make out, OCR struggles to produce accurate results. This emphasizes the need for clear and high-quality visual aids when using AI translation within virtual environments.
Interestingly, if OCR makes an initial mistake in reading text due to poor image quality, those errors can propagate through the translation process, creating larger issues down the line. The impact of a small error can be significant, highlighting the importance of good source material.
Furthermore, a constant challenge for AI translation is capturing cultural context. While AI excels at literal translations, it often falls short when it comes to understanding the subtleties and cultural implications of language. A word-for-word translation may not always convey the intended meaning or emotional tone, particularly in conversations with cross-cultural participants. This underscores a persistent gap in how well AI truly understands language within its broader context.
Another aspect that can trip up AI systems is the diversity of dialects within a single language. Someone from Spain might experience a different understanding of a translation than someone from Mexico, even though they both speak Spanish. AI struggles to differentiate between those subtle nuances, necessitating a conscious effort to choose the most appropriate translation for a particular group of users.
Remarkably, regular feedback from users can significantly improve the accuracy of AI translation models. It's quite impressive how AI accuracy can improve by 15% with consistent user input. This demonstrates the crucial role of human interaction in refining these systems. It's a beautiful interplay between humans and machines, where user feedback helps guide and enhance AI capabilities.
Some advanced OCR systems, like those developed by Microsoft, have added capabilities beyond basic translation. They also analyze the sentiment of the text, providing insights into the emotional tone of the message. This can be a game-changer in situations where conveying emotions is important.
It's also worth noting that the success of AI translation can vary significantly depending on the language pairs involved. For instance, languages with similar grammatical structures and vocabulary often translate better. English to Spanish translations tend to be more accurate than English to Japanese, illustrating how relatedness and shared language traits influence AI translation effectiveness.
The challenges for AI translation become even more apparent in real-time speech-to-speech translation scenarios. AI struggles with words that sound the same but have different meanings (homophones) and those that have multiple meanings depending on context. This can lead to mistakes and confusion, emphasizing the importance of clear and unambiguous communication when relying on AI in real-time discussions.
Finally, when deciding between AI and human translators, there's a crucial cost-versus-quality trade-off to consider. While AI translation is significantly cheaper, with prices as low as 1-5 cents per word compared to 12-30 cents per word for human translators, there's a corresponding decrease in accuracy and nuanced understanding, particularly in complex or sensitive conversations. The decision of whether to prioritize cost over accuracy is vital in choosing the right approach for a given scenario.
In essence, the pursuit of seamless, accurate parallel AI translation is an ongoing challenge, with technical and linguistic factors constantly impacting the experience. As researchers and engineers, we must continually explore these intricate relationships between AI, language, and communication to find ways to refine these exciting technologies for the benefit of improved cross-lingual interactions.
AI Translation Efficiency A Step-by-Step Guide to Managing Multilingual Zoom Sessions with Co-Hosts - Handling Audio Delay Issues Between Original and Translated Content
Ensuring smooth audio in multilingual Zoom meetings, where original and translated content are presented simultaneously, is vital to keep everyone engaged. AI translation, while generally quicker and more affordable than human translators, can introduce noticeable delays – sometimes as long as 15 seconds. These delays can break the rhythm of a discussion, especially when the conversation is fast-paced or involves quick back-and-forths. The issue becomes even more complex when you factor in dialectal variations or culturally specific phrasing, as these differences can introduce further confusion if the delay isn't managed well. Finding a way to use AI's translation capabilities while minimizing these delays is key to making these types of multilingual interactions successful. It's a balancing act between speed and the need for clear, immediate communication.
When using AI for translating audio in multilingual Zoom sessions, we often encounter a delay of 3 to 15 seconds. While seemingly short, this delay can disrupt the natural flow of conversations, especially when discussions are rapid-fire. This lag stems from the time AI systems take to process speech, translate, and then output the translated audio, showcasing that AI still has limitations in real-time processing.
The accuracy of AI translation in Zoom, particularly when relying on Optical Character Recognition (OCR) for visual content, hinges on the quality of the visuals. If the shared images are blurry or the text is difficult to discern, the initial OCR errors can propagate, leading to an inaccurate final translation. This underscores the importance of ensuring visually crisp materials when sharing content during multilingual meetings.
One of the notable limitations of AI translation is its handling of cultural context. While it excels at literal translations, it often misses the subtle cultural references and unspoken nuances that are embedded in languages. This means that a word-for-word translation might not capture the intended meaning or emotional tone, especially when navigating conversations across different cultures. It's a reminder that human intervention is still necessary to fully convey the cultural aspects of language, particularly in sensitive discussions.
AI struggles when faced with dialects within a single language. A translation perfect for a Spanish speaker in Spain might be less understood by someone from Latin America, despite speaking the same language. This issue highlights the importance of being aware of the specific audience and choosing AI translation settings or models best suited for their linguistic background.
Interestingly, AI translation models can be improved with human feedback. Studies show that consistent user input can lead to a 15% boost in accuracy. This demonstrates the ongoing role humans play in guiding and refining AI technology. The process is an intriguing dance between human and machine learning, where feedback helps bridge the gap between AI's potential and its practical use in multilingual communication.
While AI translation significantly lowers costs—often translating for as little as 1 to 5 cents per word compared to human translation's 12 to 30 cents per word—this efficiency frequently comes at the cost of nuanced understanding. This is especially apparent in intricate discussions where grasping the subtleties of language is crucial. Therefore, choosing between AI and human translators often involves balancing cost against the necessity for a deep understanding of the language and its cultural nuances.
Some of the more advanced OCR systems, like those from Microsoft, are evolving beyond simple translation and now incorporate sentiment analysis. This added capability gives the AI a deeper understanding of the context, which is essential for conveying emotions effectively in a multilingual setting.
The speed of AI translation, while impressive, can be variable. Factors like internet speed and server load affect processing times, making real-time conversation a bit unpredictable at times. These processing hiccups can introduce delays that lead to confusion and disrupt the natural flow of the conversation.
The success of AI translation can vary greatly based on the specific language pairs. Languages that share similar grammatical structures, such as English and Spanish, generally translate better than pairs with substantial structural differences, such as English and Chinese. This highlights the challenges presented by the inherent complexity and diversity of human languages.
In live, spoken translation, AI often makes mistakes with homophones—words that sound alike but have distinct meanings. This highlights the need for clarity and attention to context when relying on AI for translating spoken language in real-time. It's a reminder that even with rapid advancements in AI, careful communication is needed to ensure accurate understanding.
Ultimately, the quest for flawless AI translation in multilingual settings is a continuous process. As researchers and engineers, it's essential to continue examining the dynamic interplay between AI, language, and communication. By doing so, we can pave the way for AI translation technologies to become increasingly robust and reliable, enabling more efficient and effective cross-lingual communication in virtual environments.
More Posts from :