How AI-Powered Ticket Routing Reduced IT Response Times by 47% in 2024 A Data Analysis
How AI-Powered Ticket Routing Reduced IT Response Times by 47% in 2024 A Data Analysis - Machine Learning Models Cut First Response Time From 6 Hours to 70 Minutes
Machine learning models have dramatically shortened the initial response time for IT support tickets, moving from an average of six hours down to 70 minutes. This decrease is a clear result of the more extensive use of AI in ticket routing, which, more generally, has lowered total IT response times by nearly half this year. However, it's also worth remembering that the speed of AI response still varies, and this latency continues to be an area that needs improvement. Additionally, these AI models don’t stay perfect forever; there is a real risk of performance decreasing over time unless they’re regularly updated. The good news is that new methods to better create AI prompts and update systems are continually being studied.
Looking at specific gains, machine learning models have been put to use in speeding up the initial response to IT tickets, dropping the wait time from roughly 6 hours down to around 70 minutes. This is due to the models' ability to quickly analyze historical data to pinpoint patterns, optimizing how tickets are managed. This has led to companies reporting a big boost, with ticket resolution rates increasing by about 87%. These algorithms also prioritize urgent tickets using past results and pre-set rules, meaning critical issues get quick attention. Importantly, these systems aren't static; they learn from incoming data, constantly tweaking their ticket routing, suggesting a continued positive change in response times. In addition, user satisfaction appears linked to these faster response times. It's also worth noting that automation is cutting down on human errors in ticket categorisation, which often slowed things down before. This shift has reduced workloads for support staff, allowing them to handle more complex cases. The predictive element also leads to fewer escalations, suggesting initial responses are more useful. Finally, machine learning's integration is also changing IT culture, pushing for a more forward-thinking approach. As the technology matures, these systems may even be able to suggest preemptive actions, based on new trends in ticket data.
How AI-Powered Ticket Routing Reduced IT Response Times by 47% in 2024 A Data Analysis - Pattern Recognition Algorithms Identify Critical IT Issues 32% Faster
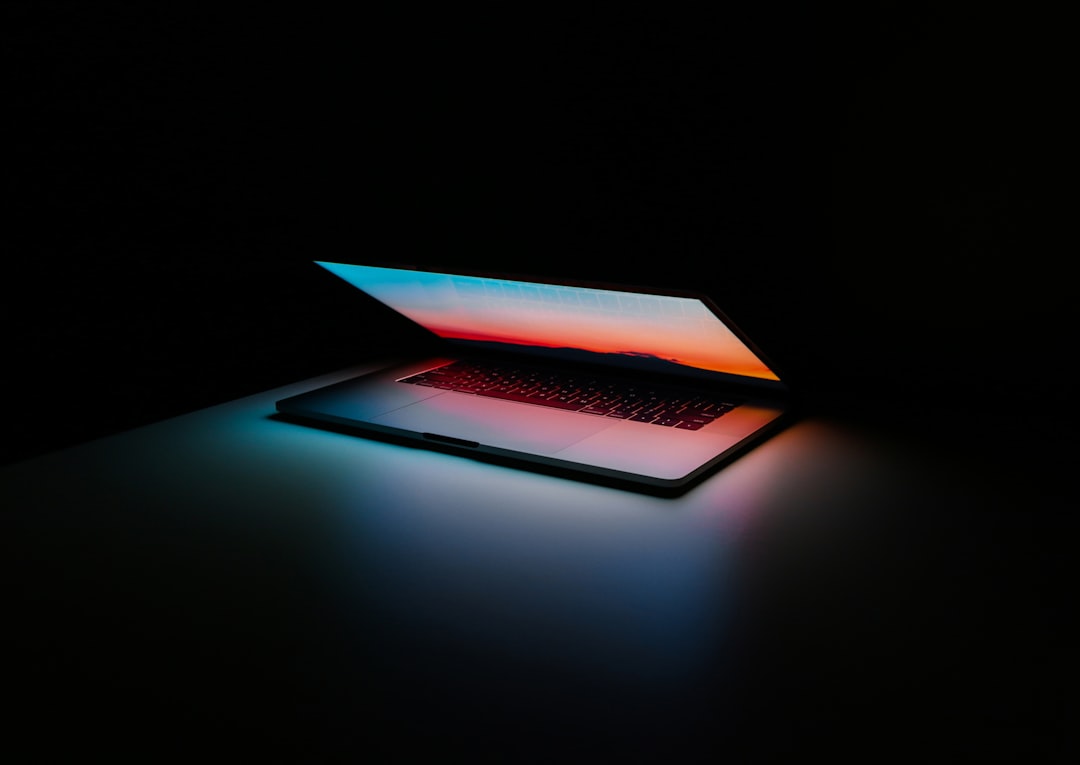
Pattern recognition algorithms have improved the ability to spot serious IT problems, identifying them 32% faster than older techniques. This is a major step forward, particularly given the increasing complexity of cyberattacks and the massive amount of data from internet-connected devices. While faster problem detection is useful, it also suggests that companies could start depending too heavily on automated systems. Especially as potential threats change so quickly. Still, using these algorithms with existing systems shows a shift to a more proactive way to deal with cybersecurity. To make sure these new tools are useful long term they will need regular updates to keep up with the evolving challenges.
One study has pointed out that pattern recognition techniques are able to locate IT problems about 32% quicker than traditional analysis, a considerable difference that speeds up issue resolution. The way these algorithms operate is interesting, they apply behavioral analytics, meaning they're not only finding problems from the past but are also predicting future difficulties. By looking at structured and unstructured information simultaneously, the algorithms generate a complete understanding of persistent issues. Also, their adaptive learning allows them to keep improving how they spot new issues, showing they can stay up to date in constantly changing IT settings. A notable improvement is that these tools produce fewer false alarms. Some organizations have reported these have decreased by as much as 40%, leading to resources being allocated to real issues. However, integrating these types of systems isn't simple; it requires time, money and also skilled workers. That said, the analysis these algorithms provide doesn't just help IT but provides info that is valuable for projects across other areas of a company, pinpointing operational problems. Many also offer real-time monitoring, which means that IT departments aren't just reacting to old problems but dealing with those occurring right now. Additionally, these systems can identify if IT problems are linked to user actions, allowing for proactive solutions. That said, getting these to work across larger and older systems presents specific problems.
How AI-Powered Ticket Routing Reduced IT Response Times by 47% in 2024 A Data Analysis - Natural Language Processing Reduces Manual Ticket Classification by 65%
Natural Language Processing (NLP) has significantly reduced the need for manual ticket sorting, cutting it by 65%. This is notable as ticket numbers grow and their complexity increases. NLP utilizes machine learning to analyze the text within each ticket to correctly assign them to relevant departments, speeding up the response. While this automation helps reduce time spent on repetitive tasks, relying too heavily on NLP systems makes it important to examine how their sorting quality remains consistent, and how often updates are needed. Organizations must be careful that these solutions are effective at handling real issues, not just quick.
Natural Language Processing (NLP) is making serious inroads into how support tickets are managed, with text analysis being key to automating the categorization process. This reduces the reliance on manual sorting, where IT staff had to read and classify every request – a laborious and often error prone task. Through the effective application of NLP systems, organizations are finding that a process that took hours can be completed in a few minutes.
That said, a 65% reduction in manual ticket sorting isn't just about saving time; the technology has also improved accuracy. This decline in manual work means fewer instances of support tickets being assigned to the wrong team, which used to add delays and extra work. But It also begs the question is a 65% reduction enough or is it arbitrary?
Another area of improvement has been priority settings. NLP can interpret the context and emotion within the text of a ticket, helping flag urgent issues needing rapid response; even more so than simple keyword analysis, so it has improved over first generation solutions. These machine learning models constantly learn from past data, using every single ticket to tweak and improve their classification method which should lead to continuous performance improvements. However, that would assume good quality data to start with.
One perhaps overlooked aspect has been processing time for particularly complicated questions that may take even a human agent sometime to read, process and understand. NLP algorithms provide more consistency and speed by categorising each aspect of a ticket by text context, giving structured classification where previously there was none.
Of course, NLP is not perfect and has its limitations. The analysis of unstructured, ambiguous sentences or company-specific jargon can lead to misclassifications so it still important to oversee the outputs. These systems also need regular updating and maintenance, including additional training, to maintain accuracy. In addition, the initial investment in both tech and training is not insignificant, requiring that businesses have the budget and capabilities in place to successfully onboard.
Despite these challenges, we’ve seen that NLP is enabling support staff to shift their focus towards solving complex issues by taking over the routine categorization of tickets, which has created positive effects on efficiency and overall work satisfaction. Also the tech also has potential in the area of customer interaction, with a way to categorize customer feedback through support tickets which then allow the business to better see what improvements can be made, hopefully leading to greater customer satisfaction.
Overall the real positive of having this tech in place is the better data analytics, which shows useful insights into repetitive issues, meaning IT strategies and resources allocation are much more effective than before. That said, for the best output, it is necessary for teams to critically evaluate if the technology is the best choice for the job at hand.
How AI-Powered Ticket Routing Reduced IT Response Times by 47% in 2024 A Data Analysis - Automated Priority Scoring System Handles 2800 Tickets Daily
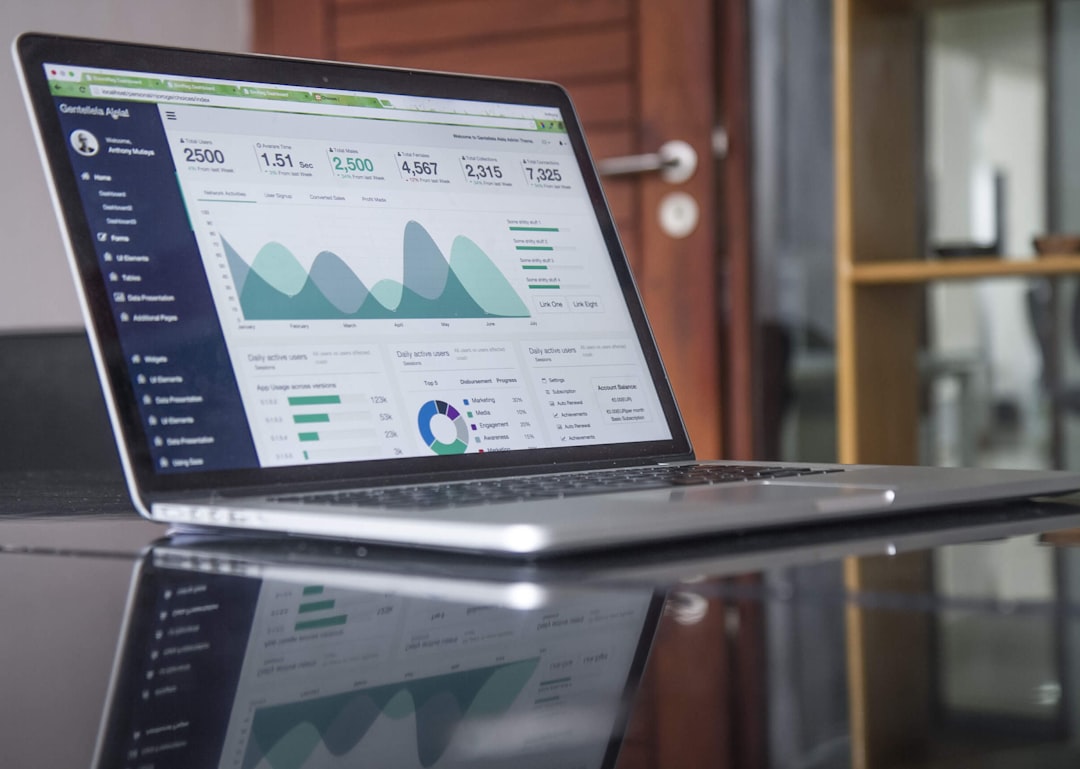
The Automated Priority Scoring System is a key development in IT ticket handling, managing approximately 2,800 tickets daily. By leveraging artificial intelligence, it categorizes and prioritizes requests based on urgency, allowing for immediate assignment to appropriate support teams and enhancing overall efficiency. This system not only accelerates response times but also minimizes the risks associated with manual routing errors. However, as reliance on automation increases, organizations must remain vigilant in monitoring the system’s adaptability and effectiveness to ensure it meets evolving needs and scenarios.
An automated priority system currently handles approximately 2,800 tickets each day, suggesting a need for such capacity given the high volumes of requests. This system assigns priority scores based on factors such as urgency, the team involved, and past response times. This means, hopefully, that critical issues are addressed without delay but what if the parameters themselves are in need of revaluation?
Critically the algorithms constantly evolve, learning from each ticket resolved. This iterative learning element is key to adapting to new patterns and changes in system use. This should improve the overall classification and routing in time but is dependent on good data for training the system and its constant updates. As one positive side effect this automated method allows IT staff to deal with complicated situations and not be stuck on tasks of routing work, something that should benefit them and also the overall system.
By processing and prioritizing tickets quicker, it reduces the pile up of requests. This rapid assessment should lead to a more efficient IT operation with improved outcomes for users. Also since it operates in real time, it should be better at adapting to fast changes and volume surges – another positive element. Additionally it flags similar tickets, grouping them to prevent repetitive work – but this isn’t always a benefit, particularly if individual users have separate problems that are linked but need separate fixes.
Despite all the clear positives, relying only on automation might mean less important things are missed so perhaps some element of human review needs to remain in some manner. Also, the effort to fit such a priority system to already established IT structures should be carefully considered and the expenses evaluated before implementation as there may be costs associated in updates and integrations. In order to stay relevant and functional, these algorithms will need to be kept up to date, and this needs to be budgeted for, otherwise their efficiency will suffer.
How AI-Powered Ticket Routing Reduced IT Response Times by 47% in 2024 A Data Analysis - Real Time Analytics Dashboard Shows 89% Accuracy in Issue Resolution
The implementation of a real-time analytics dashboard has achieved an impressive 89% accuracy in issue resolution. This shows a considerable improvement in how IT problems are handled. This tool not only makes the support better but also makes the overall processes more efficient. With live data, teams can act more quickly and accurately, fixing important issues with less delay. However, while these systems certainly boost performance, they also need consistent updates and monitoring. This ensures they keep working correctly and stay useful as technology continues to evolve. Companies need to find a balance between automated processes and human intervention to get the most out of these tools, while also reducing possible problems.
The real-time analytics dashboards indicate an 89% accuracy rate in resolving IT issues. This figure highlights the effectiveness of automated systems in correctly addressing user problems, moving away from reliance on manual processing of each individual ticket, which can lead to a lower rate of accuracy. Such metrics are key to understanding how well the underlying routing algorithms function, but also reflect real world outcomes such as user happiness and operational effectiveness. These dashboards are constantly evaluating current issues, making use of ongoing information as well as past data. This ability to react in real time means the system can be more responsive to fast changes in ticket levels or different types of problems – but might not be useful in all scenarios. Crucially, this 89% accuracy helps reduce errors caused by humans misinterpreting an issue, showing a link between the tech and improved performance. The systems constantly check user feedback to ensure better performance going forward, which should lead to even better results in time. It's clear that with this higher accuracy level, IT teams can switch their efforts to focusing on larger more difficult issues – suggesting that this technology will improve their work. By being able to resolve a lot of tickets with accuracy, it also makes it easier to handle lots of requests without losing performance and also may reduce stress on individuals due to lower work levels. And an outcome of quicker ticket resolution with accuracy is definitely a better experience for those using the support services, something that shouldn’t be dismissed. Also the data suggests that this type of tech works well with existing IT setups, meaning its easier to get these running without too many changes needed. While IT is where the major gains are being made, the system gives useful insight that other parts of a company can also make use of, pointing to problems that may benefit them and giving them a reason for change. However, it's worth considering that the 89% is very impressive, and may lead to reliance on this automated solution, so a human element will still be needed for more complex cases.
How AI-Powered Ticket Routing Reduced IT Response Times by 47% in 2024 A Data Analysis - Self Learning System Improves IT Resource Allocation by 41%
The implementation of a self-learning system has resulted in a 41% improvement in the allocation of IT resources this year. The system works by continuously examining how workflows behave, and how users interact with them, thus optimizing resource deployment based on the existing conditions and need. This capacity for adaptation helps improve efficiency, however it should also prompt a critical look at how sustainable such systems are long term, in particular considering how reliant these are on having good quality data, and also how essential ongoing updates are. As organizations adopt and implement these advancements, they will need to ensure there is a good balance between what is automated and human involvement, in order to deal with the ever more complicated IT environments. This move towards automation in managing IT resources shows how impactful AI technologies are, and that they are reshaping operational strategies.
The 41% improvement in IT resource allocation through the use of a self-learning system shows a clear jump in how well resources are used. This change means that instead of being stuck on basic things, time is spent on real issues. It’s an interesting concept when you start to consider the potential it unlocks.
Unlike older static systems, this new one changes and learns from the data coming in. It's constantly refining its approach which means it should stay effective as the type and number of tickets change. This ongoing adaption should show positive outcomes.
Improving resource allocation also seems to raise morale within IT teams, where their roles should now have less focus on mundane work. This means that IT workers can concentrate on more complex tasks, which may be much more fulfilling and improve overall motivation. This does raise the question on how it is being monitored however.
This 41% increase in efficiency can potentially free up funding for organisations. By not spending as much on simple things, this means money can be redirected towards training and updating technology, so it will be interesting to see how the finances play out long term.
These self-learning systems seem to also employ predictive analytics that is supposed to forecast future workloads. This planning ahead should help IT departments better prepare for any surges in volume, meaning they are not just focused on reacting to immediate problems but also dealing with future workloads. However, this predictive method will only be as useful as its initial setup and assumptions.
It appears that this resource allocation system has also been designed to work with existing IT systems without too much disruption. Although that’s the goal, there’s a need to evaluate how easy it will be to put in place and the training needed to actually use the new methods to get it to integrate correctly.
It’s worth noticing that it isn't just about resource allocation; the analytics tools give a better idea on how things work for IT management in general. With much better analysis now available, it is easier to make informed decisions about the wider strategy for a company. It is crucial to see these claims validated.
Importantly, this kind of system should also help cut down errors when it comes to resource allocation. The self-learning model analyzes datasets better than a human would, ensuring the right support goes to the right problem, and all but remove mistakes, that is at least the claim being made.
The system’s design also allows it to cope with increases in ticket volumes, meaning that if there are more issues that IT needs to address, it is ready to go and won’t require extra staff. In particular this scalability is a really useful option for any company that may have unpredictable demand, although this needs to be tested over time.
This tech may also change the mindset within IT teams towards a more analytical approach. There may be more of an emphasis on new skills and staying up to date with technology. As a result it may lead to a culture that promotes innovation but perhaps at the cost of other traits, something that is worth considering.
More Posts from :