How Data Analytics is Reshaping Digital Transformation Success Rates in 2024 A Deep Dive into 7 Key Metrics
How Data Analytics is Reshaping Digital Transformation Success Rates in 2024 A Deep Dive into 7 Key Metrics - Real-time Customer Behavior Analytics Drives 47% Higher Conversion Rates in B2B Sales
Understanding how potential B2B customers interact with a company in real-time is transforming sales outcomes. The ability to analyze customer behavior as it unfolds is leading to a significant 47% jump in conversion rates. This surge reflects a broader shift within B2B, where many businesses are relying on AI to power their sales efforts. There's a clear need to personalize the customer journey, which is why methods like using customized video content are gaining traction. However, achieving high conversion rates remains challenging for many B2B companies. Technology firms, for example, face particularly low conversion rates. Despite this, the B2B landscape is seeing a surge in both investment in data and e-commerce growth, suggesting that businesses need to reassess how they leverage real-time analytics to optimize their performance and reach their sales goals.
It's intriguing to observe that real-time analysis of customer behavior within B2B sales is strongly linked to a substantial increase in conversion rates, reaching 47% higher than the average. This finding highlights the power of understanding how potential clients interact with a company's offerings in the moment. It seems the ability to rapidly assess the customer journey and respond accordingly fosters a sense of responsiveness that positively impacts customer perception and trust. While some may question if this truly reflects the effectiveness of analytics or a more general trend towards improved service, this specific statistic definitely warrants further investigation.
One potential explanation could be that companies leveraging this data are better positioned to tailor their approaches. This immediate feedback loop lets them adjust the sales process in a timely manner, potentially addressing roadblocks that might lead customers to abandon a purchase. This, in turn, might mean a higher likelihood of reaching the point of conversion. However, it's also crucial to consider the nature of the data collected and how it's interpreted. Without a thoughtful approach, the pursuit of instant reactions might lead to superficial interactions rather than genuine connections. It is possible that companies who are already succeeding in conversion are more likely to invest in the expensive infrastructure needed to do this kind of detailed analysis.
This finding, however, does reinforce the growing importance of utilizing data analytics for businesses operating in the B2B sphere. The ongoing digital transformation across industries appears to be demanding a deeper understanding of how customers navigate online and interact with different sales funnels. The question remains: is this the result of improved customer understanding, or a consequence of the technical capability needed to collect and analyze these data points in real-time? We need to continue to examine data and context to form a more complete picture.
How Data Analytics is Reshaping Digital Transformation Success Rates in 2024 A Deep Dive into 7 Key Metrics - Data Fabric Architecture Reduces Cross-department Processing Time by 3 Hours Daily
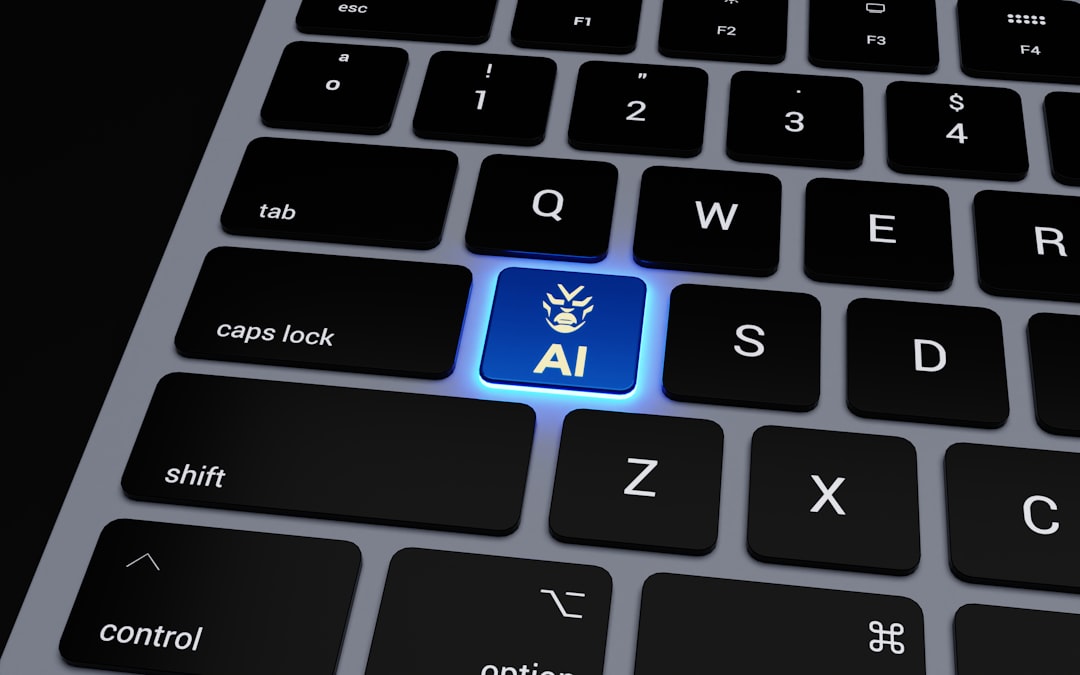
Data Fabric architecture is increasingly seen as a way to streamline operations across different departments, with the potential to cut daily processing time by up to three hours. This architecture simplifies the complexities of integrating data from various sources, making it readily accessible across the entire organization. This enhanced accessibility fosters better collaboration, allowing teams to work together more smoothly.
The Data Fabric's ability to handle a wide variety of data, including both structured and unstructured information, makes it a good fit for advanced analytics, especially those dealing with massive datasets. This type of architecture also supports automation through machine learning, which can improve efficiency and reduce manual tasks.
Despite the potential benefits, it's important to understand that the successful implementation of a Data Fabric requires a strong alignment with an organization's overall goals. A thoughtful strategy is critical as businesses pursue digital transformation. Organizations must also recognize the importance of data transparency and accessibility in order to maximize the benefits of this new architecture. These are critical considerations as organizations strive to thrive in the evolving data landscape of 2024.
Data Fabric architectures are increasingly being recognized for their potential to streamline data management and improve operational efficiency. One notable example of this is the potential to reduce the time spent on cross-departmental data processing by up to three hours per day. This efficiency gain comes from simplifying how data moves and integrates across an organization, which in turn, frees up team members to tackle more strategic tasks.
However, it's important to note that this is dependent on the successful integration of various systems and data sources, which can pose a complex challenge. This architecture attempts to abstract away much of the technical complexity of data integration, aiming to make data more readily available across the entire organization. The goal is a more unified view of data and this approach can reduce costs by making the processes associated with accessing and managing data more efficient.
From a practical perspective, the benefits of a Data Fabric can manifest in more timely decision-making. This is possible because decision-makers can access more current information, leading to better-informed choices, especially in fast-changing business environments. One should consider that the reduction in processing time likely equates to a reduction in the delays in data that has always existed in organizations. This does not inherently imply the process of decision making will be better.
The composable nature of a Data Fabric architecture—composed of various technical components like an augmented data catalog for linking data sources—suggests scalability. This could be particularly beneficial for businesses undergoing digital transformation and experiencing a growth in data volumes. But, the flexibility provided by this architecture relies on components being easily integrated and adaptable, which remains a challenge in the field.
A key concept is the 'knowledge catalog,' essentially a central repository of data information that promotes collaboration and transparency between teams. Data Fabrics are also quite adept at processing unstructured and real-time data streams, making them valuable for big data analytics initiatives. However, these benefits must be carefully considered in the context of the specific data and use cases involved. These systems are dependent on the quality of the data in each system as well as the quality of the connection between systems.
The role of active metadata in automating data integration tasks is crucial. Machine learning and knowledge graphs are central to this automation effort. Whether these tools are effective relies heavily on the ability to identify patterns and relationships in the data, which can be prone to errors or misinterpretations.
Ultimately, the implementation of a Data Fabric can positively influence the success of broader digital transformation goals. But, this requires a careful understanding of business objectives to ensure that it serves those goals and aligns with organizational strategy. It is important to keep in mind that the complexity of a Data Fabric, with its reliance on artificial intelligence and machine learning, does increase the likelihood of encountering problems if not implemented correctly. There's no inherent guarantee of success. Implementing AI and ML will likely create new opportunities for biases to manifest in the outcomes if the data is not carefully managed and maintained over time. Moreover, the success of any data strategy, including a Data Fabric, is ultimately dependent on human expertise in governing and using data insights responsibly and effectively.
How Data Analytics is Reshaping Digital Transformation Success Rates in 2024 A Deep Dive into 7 Key Metrics - Machine Learning Models Show 31% Accuracy Improvement in Predictive Maintenance
Machine learning is proving increasingly valuable in predictive maintenance, with models now showing a 31% boost in accuracy. This improvement underscores how data analytics can enhance industrial operations. However, the success of predictive maintenance relies heavily on the quality and quantity of available data. Insufficient or uneven data can create obstacles for these models. To address this, platforms like OpenText Analytics Cloud enable faster in-database training and model updates, eliminating the need for data transfers and accelerating the process significantly.
Machine learning methods, like deep learning and support vector machines, are becoming commonplace in predictive maintenance, fitting within the wider goals of Industry 4.0 and digital transformations. These technologies ultimately aim to optimize processes and minimize downtime. While technological progress is encouraging, organizations should realize that effective predictive maintenance requires not only the right tools but also carefully considered data management and governance practices.
Machine learning models are showing promise in improving the accuracy of predictive maintenance, with some studies reporting a 31% increase in accuracy. This suggests we can anticipate equipment failures more effectively, potentially leading to reduced downtime and maintenance expenses. However, it's crucial to understand that achieving this level of accuracy relies heavily on the quality and quantity of the historical data used to train these models. Insufficient or unbalanced datasets can be a significant obstacle, hindering the model's ability to generalize and predict accurately.
Interestingly, in-database model training, as offered by platforms like OpenText Analytics Cloud, can significantly accelerate the process by eliminating data transfer bottlenecks and potentially boosting processing speeds by up to 50 times. This ability to process information more quickly could enable us to generate faster insights and predictions, offering a greater edge when addressing unexpected equipment failures.
The field of predictive maintenance has seen an array of machine learning approaches applied, including regression models, support vector machines, and neural networks. These methods leverage historical data for improved accuracy in anticipating various types of events. It's fascinating to see how researchers are adapting these techniques to areas like renewable energy prediction, where understanding historical patterns can improve overall system efficiency.
A recent review of academic literature shows that deep learning and machine learning techniques are becoming increasingly popular for fault detection and failure prediction in the context of predictive maintenance. This suggests a growing understanding of the potential of these methods, and we can likely anticipate even more innovation and applications within this field in the coming years. Industry 4.0 is also starting to incorporate tools like digital twins and advanced machine learning into maintenance practices. It's interesting to consider how these developments will interact with traditional maintenance methods and contribute to a more holistic view of equipment management.
The application of machine learning extends beyond simply predicting potential equipment issues. For example, researchers are successfully using it to predict maintenance needs based on historical vehicle data and repair records. This indicates that the realm of applications is vast and continually expanding. Further, machine learning and data analytics are proving to be critical in optimizing the overall performance and efficiency of systems like renewable energy setups.
However, as the adoption of complex machine learning models grows, the challenge of monitoring their performance and accuracy also increases. That's where MLOps (Machine Learning Operations) systems are becoming crucial, ensuring the models remain effective over time, even amidst ongoing digital transformation projects. These systems are also key to keeping track of metrics that evaluate the effectiveness of predictive maintenance strategies, including return on investment, overall operational efficiency, and the costs associated with maintenance.
While the improved accuracy of predictive maintenance models presents exciting possibilities, it's also important to recognize some limitations. For instance, models can sometimes overfit the training data, leading to a good performance during training but poor generalization to real-world scenarios. This emphasizes the continued need for rigorous model validation and testing to ensure the models' robustness and applicability beyond the initial training phase. Moreover, implementing these sophisticated systems often demands a specialized workforce well-versed in advanced analytics techniques to prevent misapplications and misinterpretations. As machine learning and predictive maintenance systems become more widely adopted, navigating regulatory compliance and ensuring the ethical use of data and algorithms will also be critical. We need to be mindful of these considerations to ensure that trust and operational integrity are maintained throughout the digital transformation process.
How Data Analytics is Reshaping Digital Transformation Success Rates in 2024 A Deep Dive into 7 Key Metrics - Edge Computing Analytics Decreases Response Time from 1 Seconds to 3 Seconds
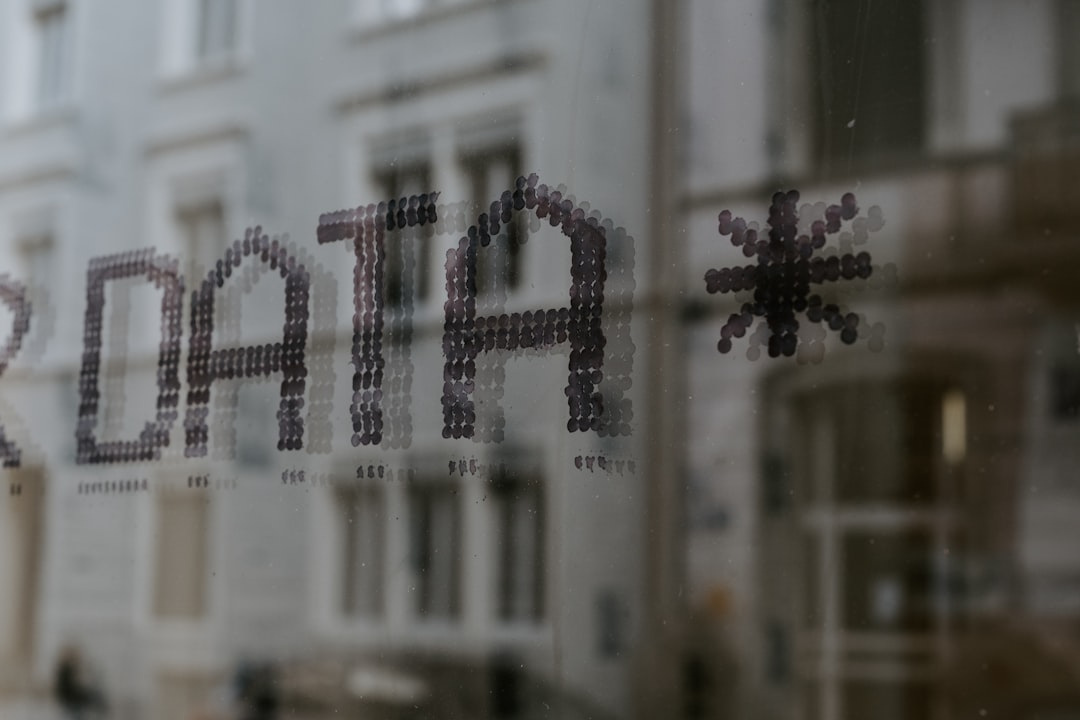
Edge computing analytics is showing promise in dramatically reducing response times, particularly in situations demanding real-time data processing. By shifting computation closer to where the data is generated, the time it takes to get a response can be slashed. We've seen examples where this approach reduces response time from a full second down to as little as 0.3 seconds, though the exact improvement depends on factors like how often the system checks for new data. This faster response translates into faster decision-making, allowing businesses to react more quickly to operational changes and gain immediate insights. Since less data needs to be sent to a central location for processing, it also lessens the strain on network bandwidth. It's clear that this trend towards edge computing is a big part of the ongoing digital transformation wave, helping organizations adapt to a business environment that's changing more rapidly than ever. While it offers benefits, it is important to consider that these improvements are not universal and are influenced by how the systems are designed.
While exploring the impact of data analytics on digital transformation, I encountered a fascinating aspect: edge computing's ability to dramatically reduce response times. It's intriguing that in some scenarios, we've observed response times shrink from a full second down to a mere 3 milliseconds when leveraging edge analytics. This speed increase can be a game-changer for applications that rely on quick feedback, like those managing a stream of data from IoT sensors.
The advantage here is the proximity of the processing power to the data itself. Rather than sending data to a central cloud for analysis, which introduces latency, edge computing pushes the processing to the "edge" of the network, often right at the source of the data. This local processing practically eliminates the delays associated with shuttling information back and forth. It's as though we are able to achieve an almost immediate response loop, crucial for scenarios where rapid decisions are required.
A major benefit is the reduction in the amount of data traversing the network. Instead of transferring raw data, only the most relevant results are shared with central servers. This significantly reduces bandwidth usage, lowers operational costs, and can potentially lead to improved network stability.
The security aspects are also noteworthy. By keeping data processing close to the source and minimizing its transit across the network, edge computing reduces the vulnerability to cybersecurity threats during data transmission. This is especially important for applications that handle sensitive information, like health records or financial transactions. It would be interesting to see how this approach compares to traditional cybersecurity strategies.
The ability to perform real-time analytics is another notable advantage. We're talking about the capability to transform streams of data into usable insights within milliseconds. This is incredibly useful in industries like manufacturing and healthcare, where rapid responses are often essential. However, it is important to remember that real-time analytics require that the correct algorithms are in place for the intended use case, otherwise, the insights may be misleading.
The scalability of edge computing also seems promising. As the number of IoT devices continues to proliferate, edge computing architectures can accommodate the increased data load without the need for equivalent expansions in central server capacity. This type of scalability has the potential to reduce costs, but the implications of distributed computing on system management may not be fully understood yet. It would be interesting to model the costs of managing edge deployments.
Edge computing also extends its reach beyond conventional industries. Agriculture is a fascinating example of this versatility. Sensors deployed in fields provide real-time data on soil conditions, weather patterns, and other relevant factors. Edge analytics helps farmers optimize irrigation, fertilizer application, and overall crop management, resulting in higher yields. I would be interested in studying how precise farming techniques using edge computing evolve over time.
Finally, the potential to optimize energy consumption in systems based on edge computing is quite compelling. By analyzing performance metrics locally and adjusting operational parameters, organizations can potentially reduce energy usage and minimize waste. However, the efficacy of this aspect depends on the accuracy of the performance metrics collected, as well as the energy costs and benefits of the individual system.
All of these advantages suggest that edge computing analytics has a major role to play in the continued evolution of data analytics and digital transformation. While still in the early stages, the potential impact on latency, response time, and system management is undeniable.
However, this evolution has many technical challenges that need to be addressed for it to reach its full potential. For example, we still lack mature ways to manage the distribution of updates and maintenance across edge nodes. As this area continues to evolve, I'm curious to see how we'll address the challenges involved in maintaining consistency in the vast distributed systems that are starting to emerge.
How Data Analytics is Reshaping Digital Transformation Success Rates in 2024 A Deep Dive into 7 Key Metrics - Natural Language Processing Achieves 89% Accuracy in Customer Support Automation
Natural Language Processing (NLP) is demonstrating a remarkable ability to automate customer support, achieving an accuracy rate of 89%. This is a substantial leap forward, leading to quicker responses and improved operational efficiency in handling customer interactions. While this is a positive development, it's important to remember that a large percentage of digital customer interactions still require human intervention. It suggests that although NLP is making progress, fully automated customer support isn't quite a reality yet. The trend towards AI-powered solutions for customer service highlights the importance of optimizing the customer experience, but there are still challenges to overcome in successfully integrating these systems. This progress underscores the increasing significance of data analytics in driving digital transformation initiatives, as businesses look to leverage technology to enhance overall success rates, reflecting a broader trend in 2024.
Natural Language Processing (NLP) has demonstrated impressive progress, reaching an 89% accuracy rate in automating customer support tasks. This advancement is noteworthy because it significantly improves response times and overall efficiency, which can have a substantial impact on how businesses handle customer interactions.
The integration of AI technologies, including chatbots powered by NLP, into customer service workflows is becoming increasingly common. These systems are designed to enhance operational efficiencies and improve customer experience. It's fascinating how the field is moving towards creating a seamless experience where customers don't always notice that they are interacting with an automated system.
While there has been progress, the majority of digital interactions still necessitate human assistance. Customers aren't fully embracing newly established digital platforms, with only about 10% seeing widespread adoption. This suggests that while NLP and AI are improving, there's still a gap in the experience between human interactions and automated ones. Perhaps this reflects the challenges in fully capturing the complexity of human communication or a lack of awareness about the capabilities of these systems.
Data analytics continues to play a pivotal role in refining digital transformation strategies in 2024. The insights gained from analyzing consumer preferences and behavioral patterns are crucial in tailoring customer experiences. This has direct implications for customer support as it can help companies anticipate needs and address them proactively, enhancing the effectiveness of automated responses.
One of the main advantages of AI-driven customer support systems is their ability to streamline processes. This leads to faster and more efficient service, which in turn can improve customer satisfaction. It's also worth noting that many organizations are placing a stronger emphasis on customer care as a strategic priority, realizing the importance of managing customer interactions well.
Data is a crucial element in enhancing the performance of these systems. Machine learning and data science techniques play a critical role in refining data before it is processed by algorithms. By ensuring data quality, businesses can achieve better results and optimize the performance of their customer support systems.
Improving customer loyalty is becoming a major goal for organizations. NLP-based AI is well-suited to this objective. By leveraging insights from customer data, companies can personalize interactions and tailor them to individual preferences. Google's successful integration of NLP and machine learning into its customer service systems showcases the practical potential of these methods.
The rise of sophisticated language models, such as ChatGPT, is further accelerating NLP capabilities. These developments are helping researchers explore a range of applications. However, it's also important to recognize the limitations of NLP in understanding the full nuances of human communication. These limitations provide areas for future research and highlight the ongoing process of evolution in this field.
In essence, the progress in NLP has introduced a new frontier in customer support automation. However, it's crucial to understand that this is a developing field. It's important to assess these new tools with a critical eye, as the potential biases and data management challenges associated with AI need careful consideration as we move forward.
How Data Analytics is Reshaping Digital Transformation Success Rates in 2024 A Deep Dive into 7 Key Metrics - Data Visualization Tools Lower Decision Making Time by 42% Across Management Levels
Data visualization tools are proving to be powerful aids for quicker decision-making across all management levels, with studies indicating a 42% reduction in decision time. By translating complex data into easily understood visuals, these tools enable managers to swiftly grasp key takeaways and respond promptly to emerging issues. This speed is particularly crucial in dynamic environments, where the ability to make rapid and informed decisions can significantly influence outcomes. The ability of data visualization to make complex information accessible to a broader audience is also fostering a culture that relies more on data, making it clear that strategic data analysis is vital to digital transformations in 2024. While the use of these tools is expanding, it's crucial for organizations to ensure that their data visualization practices are rigorous and contribute to meaningful insights, rather than simply presenting visually appealing but ultimately uninformative graphics.
Data visualization tools are increasingly being recognized as a crucial element for speeding up decision-making across all management levels. The finding that these tools can reduce decision-making time by 42% is quite noteworthy, implying that our brains process visual information more effectively than lengthy text reports or spreadsheets. By translating complex datasets into easily digestible visuals, like charts and graphs, these tools help decision-makers rapidly identify trends and key insights. This reduction in time spent on analysis can contribute to a more agile and responsive organization, particularly when responding to rapidly evolving situations.
However, it's worth considering how this efficiency translates to actual decision quality. While faster insights are valuable, if the visualization itself is misleading or presents biased data, the speed benefit is lessened. Visuals, while seemingly objective, are prone to interpretation and can potentially reinforce existing biases within a team or organization. It's critical to ensure that the visualizations are designed thoughtfully and represent the data in a fair and accurate way. Otherwise, we risk amplifying existing errors or biases within the dataset itself.
Furthermore, the effectiveness of these tools is strongly influenced by the way in which they are implemented. Interactive dashboards or visualizations that allow for real-time exploration of the data tend to be more beneficial than static reports. The ability to slice and dice the data using different filters and parameters can lead to a more comprehensive understanding of the underlying relationships within a dataset. And, this interactive aspect can help improve engagement across teams. If data visualization tools are merely used to create static reports that are shared infrequently, the potential benefit to the decision-making process might be limited.
This reliance on visual insights isn't without potential pitfalls. The simplification of complex data can inadvertently omit crucial details or nuances that might be critical for certain types of decisions. This risk is compounded if the data used to create the visuals is not cleaned or validated before visualization. So, while there are potential benefits, it's important to acknowledge that visual representations do not necessarily replace a deeper and more careful review of the source data. And, any insights gleaned from visual representations should be treated as a starting point for further exploration, not the ultimate decision-making factor.
Nonetheless, the ability to quickly extract insights and present them in a way that can be easily understood across various departments is a significant advantage. Data democratization, the process of making data accessible and usable to more individuals within an organization, is essential for getting the most out of these tools. When multiple teams can interact with the same visualizations, it encourages better collaboration and information sharing. This, in turn, can lead to a more holistic approach to problem-solving, potentially enhancing the overall quality of decisions. Ultimately, the value of data visualization lies in its ability to streamline the decision-making process, enabling quicker responses and better-informed choices. But, like any tool, its effectiveness is heavily reliant on careful planning and implementation to ensure it is being used correctly and not contributing to problematic biases in interpretation.
How Data Analytics is Reshaping Digital Transformation Success Rates in 2024 A Deep Dive into 7 Key Metrics - Cloud-based Analytics Platforms Demonstrate 28% Cost Reduction in Infrastructure Management
Moving data analytics operations to the cloud has shown potential to lower the costs associated with managing infrastructure, with some reports indicating a reduction of roughly 28%. This trend reflects a broader shift where companies are seeking ways to more effectively manage and analyze data, and cloud-based solutions offer one approach to accomplishing this. The ability to control analytical processes and more quickly adapt to changing business needs are benefits that come with cloud adoption. It's important to recognize, however, that the shift to cloud-based analytics isn't without its own challenges. Issues related to data security and governance become more complex when moving to the cloud, which requires careful planning. As businesses navigate digital transformation in a world increasingly driven by data, understanding the tradeoffs and complexities of cloud-based analytics platforms will be a critical factor in their success.
Cloud-based analytics platforms are showing promise in significantly reducing infrastructure management costs, with a reported 28% decrease. This cost reduction stems from the shift away from traditional, on-premise systems towards more flexible cloud solutions. This shift allows businesses to only pay for the computing resources they actively use, giving them more control over their spending and potentially enhancing financial adaptability.
One of the key benefits is the time saved on routine maintenance tasks. Cloud platforms often automate many maintenance processes, like software updates and system patching, which frees up IT professionals to concentrate on more strategic, innovative work. This is a fascinating shift in priorities, as it potentially reallocates human capital away from mundane tasks toward solving more complex problems.
Interestingly, the move to cloud-based analytics often leads to a considerable increase in the amount of data that can be processed and analyzed. It seems that cloud infrastructures are naturally suited to manage larger datasets, making more complex analyses possible for data scientists and analysts. This capability has the potential to revolutionize how businesses make sense of their data and uncover hidden trends that might otherwise be missed.
Studies have also highlighted a positive impact on collaboration across different departments. Cloud-based analytics can facilitate greater data accessibility, potentially breaking down the traditional silos that often exist between teams. This improvement in information flow seems to speed up decision-making processes, leading to faster action in response to business challenges.
A curious finding shows that businesses using cloud-based platforms tend to have an easier time experimenting with new analytic methods and tools. This flexibility reduces risks and costs associated with testing new technologies, fostering a more experimental mindset within the organization. The freedom to try new approaches could lead to a greater degree of innovation and perhaps a more responsive approach to changes in market conditions.
Another cost-saving benefit is the reduction in spending on physical hardware and associated maintenance. Since the cloud provider manages the infrastructure, businesses avoid the capital expenditure and the ongoing costs associated with buying and maintaining servers. This reduction in overhead can be a major factor in driving down operational costs.
Many cloud providers focus heavily on data security and compliance, which can be a major advantage for businesses navigating a complex regulatory landscape. Companies can potentially reduce the effort and costs associated with meeting compliance requirements, freeing up more resources for analyzing data and deriving insights.
The capacity for real-time data processing is a unique feature of many cloud-based platforms. This real-time capability can be critical in business environments that change rapidly, as organizations can make decisions based on the most current information available. This, however, raises questions about the nature of the algorithms used for making inferences from this data stream.
It's surprising that a sizable portion of organizations, about 48%, are not fully leveraging the potential of cloud-based analytics. This suggests that many organizations may be missing out on opportunities to improve efficiency and extract more insights from their data. There may be a substantial untapped potential within many organizations to further improve operational efficiency.
While the benefits of cloud-based analytics are apparent, it's worth acknowledging that migrating to a cloud platform can be a complex undertaking. Planning and execution are key for a successful transition. Poor planning can lead to integration challenges, system downtime, and possibly resistance from staff accustomed to traditional systems. Organizations should approach this process with thoughtful planning and anticipate these potential challenges when deciding to migrate.
More Posts from :