Salesforce Marketing Cloud 7 Key Advancements in AI-Driven Personalization for 2025
Salesforce Marketing Cloud 7 Key Advancements in AI-Driven Personalization for 2025 - AI-Powered Real-Time Messaging Across Multiple Channels
In the near future, the ability for businesses to engage with customers in real-time across numerous communication channels is poised to dramatically change how marketing operates. Salesforce Marketing Cloud's integration of AI allows for dynamic optimization of marketing campaigns, tailoring messaging based on each individual's preferences and actions. This is achieved by using the system's built-in predictive AI to adapt and refine campaign delivery across channels like email, social media, and mobile apps.
The core of this approach is understanding customer behavior and intent, fueled by a company's own customer data. This focus on first-party data allows for a more nuanced grasp of individual needs and preferences, enabling more effective engagement strategies. However, implementing these AI-powered real-time systems won't be without hurdles. Companies must manage data efficiently and navigate the complexities of integrating these advanced technologies into their existing operations.
Ultimately, the potential for AI-driven real-time messaging across channels is considerable, promising a personalized experience that could profoundly alter customer interactions. While there are hurdles to overcome, brands that successfully embrace this technology will likely see improvements in marketing ROI and customer satisfaction.
Salesforce's push towards real-time AI-driven messaging across multiple channels is an interesting development. The idea that AI can sift through vast amounts of data – potentially over a million data points each second – to instantly adjust messaging based on a user's actions is certainly impressive. It's intriguing how these systems can adapt so rapidly, leading to potentially higher engagement rates, as some studies suggest a 20% increase.
However, the concept of AI predicting customer inquiries before they're even sent is both fascinating and slightly concerning. While the use of historical data for such predictions can improve response times and customer satisfaction, it also raises questions about user privacy and the potential for bias in the algorithms. Maintaining a consistent brand voice across multiple channels with AI is certainly a challenge, but potentially also a significant advantage. It's remarkable that AI can ensure messaging harmony across email, SMS, and social media, though I imagine the nuances of language and tone for each platform would be complex to master.
The promise of predictive analytics for tailoring messaging strategies is tempting, potentially leading to increased conversions. But, it's vital to remember that the consumer expectation of personalization is widespread – about 70% anticipate it. This creates pressure to deliver on the promise, which is further complicated by the need to be mindful of privacy and avoid any sense of intrusion. The rise of AI-powered chatbots is undeniable, and handling 80% of basic queries autonomously can free up human agents for more complex issues.
However, this effectiveness is also linked to the potential risks. If the AI isn't carefully configured, there's a chance for miscommunication and inconsistency, which could seriously erode customer trust and damage a brand's reputation. It is important that the human element be factored into the design of such systems, and not just seen as a means of reducing human interaction. Finally, the notion of drastically reduced response times – down to mere seconds – is another powerful benefit of AI-powered systems. This real-time responsiveness can create a competitive advantage in today's fast-paced markets. Yet, the question of what happens when these systems encounter unforeseen situations, or errors in their processing needs to be considered.
Salesforce Marketing Cloud 7 Key Advancements in AI-Driven Personalization for 2025 - Enhanced Data Analytics for Predicting Customer Behavior
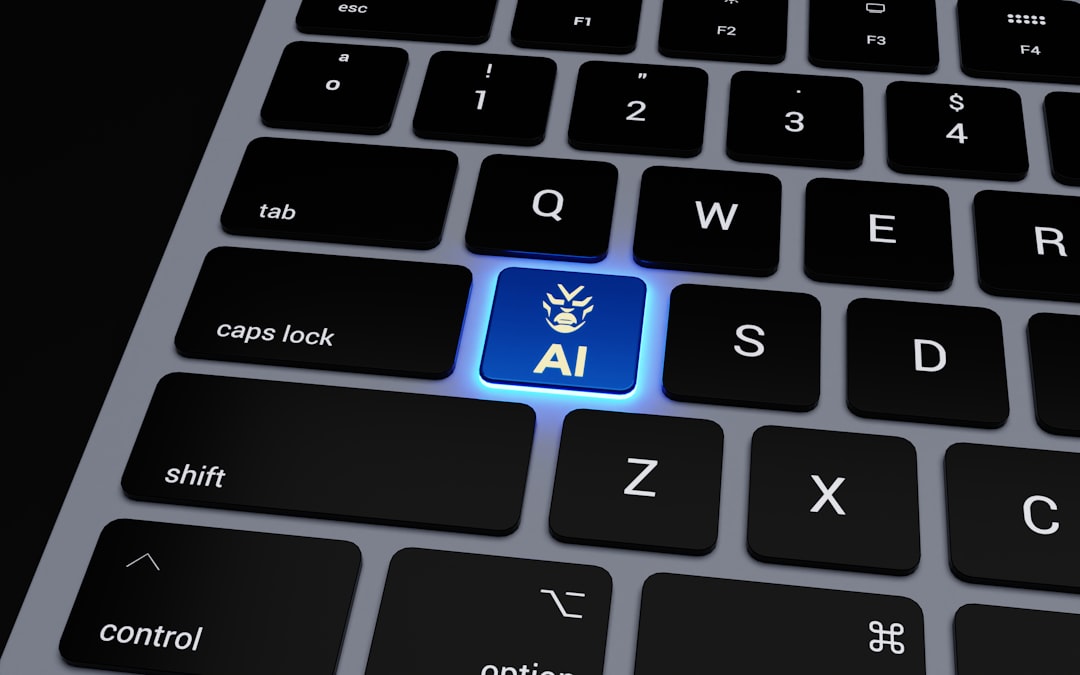
Enhanced data analytics plays a crucial role in helping businesses predict customer behavior and tailor their marketing efforts. Salesforce Marketing Cloud incorporates sophisticated AI capabilities to analyze vast amounts of customer data, allowing for more accurate predictions of individual preferences and actions. This increased understanding of customer behavior enables companies to design personalized experiences and develop more impactful engagement strategies. However, the transition towards this AI-powered approach is not without its complexities. Businesses need to find ways to manage the potential risks associated with handling sensitive customer data and integrating these new AI systems into their existing operations without disruption. As consumers increasingly expect personalized interactions, companies face the challenge of meeting those expectations while adhering to data privacy regulations and avoiding any sense of intrusiveness. Ultimately, the future of effective customer engagement hinges on effectively navigating these challenges and leveraging the power of data to create meaningful connections with customers.
Salesforce Marketing Cloud's advancements in AI aren't just about sending messages at the right time, they're increasingly about digging deeper into customer data to better predict behavior. We've seen that integrating various data sources, like purchase history, website interactions, and even social media activity, can significantly boost the accuracy of predicting how a customer might act. Some researchers suggest a potential 50% increase in accuracy with this approach. It makes sense—the more information we have, the better we can understand what drives a person's decisions.
This also leads to improved estimations of customer lifetime value. I find it fascinating that some machine learning models can forecast this value with such precision, often within just 5% of the customer's true worth. Imagine being able to pinpoint your most valuable customers with that kind of accuracy—it would certainly change how resources are allocated.
But it's not just about identifying valuable customers. The context of a customer's interaction matters too. Personalizing a message based on where someone is or what they've recently viewed can increase engagement dramatically. We're talking about a 30%+ jump in response rates—that's a powerful incentive to implement these techniques. It's interesting to think how understanding the 'when' and 'where' can shift how effective a marketing campaign is.
We can go further than just predicting engagement. Sentiment analysis, which essentially uses natural language processing to gauge emotions in customer feedback, allows businesses to understand if someone is happy or frustrated. I think that being able to proactively address concerns or praise satisfied customers is a big improvement over reacting only to obvious problems. It’s much more effective and makes customers feel valued.
It's not just about predicting satisfaction—we can even try to foresee if someone is about to leave. It's surprising how well some models can identify the risk of customers churning, or canceling a service. The opportunity to intervene before they leave is appealing, as research shows it can reduce churn rates by as much as 25%. It's like being able to spot potential problems before they emerge.
One of the big advantages of enhanced data analytics is the ability to simulate various marketing scenarios. This means we can test out ideas in a virtual environment and see what might happen before spending money on a campaign that might flop. The potential for reducing wasted marketing funds by up to 20% is compelling—the ability to avoid expensive mistakes is valuable.
But the speed at which AI can process information is equally remarkable. We're talking about analyzing millions of transactions in seconds, allowing companies to get insights quickly and adjust accordingly. The implications for faster decision-making are huge. It's a bit like having a crystal ball for business trends.
Furthermore, by focusing on the most relevant segments and messaging, businesses are seeing a significant increase in marketing return on investment (ROI) – in the range of 15-20%. This seems to show that accurately forecasting behavior, and acting on that information, can be a powerful tool for improving the effectiveness of marketing campaigns. It’s impressive how a targeted approach can improve the bottom line.
However, the ability of these algorithms to react to changes in the wider environment—like economic downturns or shifts in cultural trends—is perhaps the most exciting aspect. It suggests we can have marketing strategies that adapt in real-time. It’s fascinating how this dynamic approach might allow businesses to be more responsive to change and ultimately improve their position in a constantly evolving marketplace.
And this speed even gives us the ability to spot trends before they become widely recognized. It's almost like being able to see the future of customer preferences. This ability to be a market innovator by anticipating needs is very interesting and suggests a new way to interact with customers and be more successful in the market. It's definitely an area worth watching in the coming years.
Salesforce Marketing Cloud 7 Key Advancements in AI-Driven Personalization for 2025 - Integration of Marketing Cloud with Data Cloud for Improved Data Management
The merging of Salesforce Marketing Cloud and Data Cloud signifies a noteworthy shift in how businesses manage customer information. By integrating these two platforms, organizations gain access to a more complete picture of their customers across various touchpoints, like marketing campaigns and other interactions. This comprehensive view allows for smarter decision-making and a greater ability to tailor interactions to individual preferences. The automation of workflows and personalization of customer journeys is also significantly enhanced through this link. To ensure a smooth integration, Salesforce suggests using a specific Marketing Cloud API user for authentication. This approach prioritizes secure data management and minimizes potential access issues. Overall, the integration offers a more unified and powerful approach to customer interaction management, paving the way for a more responsive and personalized customer experience. While this integration provides benefits, it is important to acknowledge potential pitfalls in maintaining data privacy and ensuring seamless transition into existing systems.
The linking of Salesforce's Marketing Cloud with its Data Cloud presents some fascinating possibilities for managing customer information more effectively.
Firstly, it provides a more complete picture of each customer. By pulling data from a wider range of sources, including sales, service, and even commerce interactions, businesses can build a much richer profile of individuals. This richer profile can improve customer segmentation, making it possible to tailor marketing efforts more precisely. While this improved targeting has the potential to increase marketing impact, it’s worth considering how this comprehensive view of customers might impact individual privacy.
Furthermore, the combination of these two systems seems to enable a shift towards real-time data processing. This ability to process data as it comes in is crucial for marketers looking to respond rapidly to customer interactions, like a visit to a specific part of a website or a social media post. The question is whether real-time adjustments are always the best approach. There may be instances where a slightly delayed response might allow for a more thoughtful and considered action.
The integration also appears to leverage AI in interesting ways. By combining historical interactions with AI prediction models, it's possible to create more accurate forecasts of future customer behavior, potentially boosting the success rate of predictions by a considerable margin. While these improved predictions are attractive, I am curious about the extent to which these AI models incorporate a diverse range of individuals and how well they avoid any biases that might exist within the data they are trained on.
One interesting aspect is the concept of "dynamic segmentation". This refers to the ability to automatically group customers into different categories as their behaviors change, allowing for much more agile marketing. I'm curious about the practicality of implementing this for a business, as the algorithms used for segmentation must be carefully tuned to ensure they effectively meet business goals without alienating customers.
It’s also worth noting that this integration might be beneficial for maintaining a consistent brand voice across different communication channels. A common problem for companies is making sure that the brand's personality and message stay the same whether a customer is interacting through email, social media, or a mobile app. The integration of Marketing Cloud with Data Cloud appears to offer a method for streamlining this, although ensuring that messaging is effective across platforms with unique characteristics remains a challenging task.
The combination of the two clouds also seems to improve the ease of staying compliant with data privacy rules. By centralizing and managing data more efficiently, businesses may be able to handle regulations like GDPR more readily. This is a vital aspect of responsible data management and shows the potential for minimizing the risks associated with data breaches or mishandling.
In addition, the integration seems likely to increase the automation of marketing processes, leading to streamlined workflows and freeing up teams for tasks that require more human judgment. This increased automation, however, raises questions about the role of human oversight and how marketers ensure these automated systems do not become overly simplistic or insensitive in their communication.
Another positive point is the potential for reducing the errors that can occur during data entry or processing. By establishing a centralized data repository, it becomes easier to avoid inconsistencies and use data that is known to be accurate.
The enhanced analytical tools made available through this integration are also worth mentioning. Marketers can access a wider array of analysis and gain deeper insights without a great deal of manual effort. This access to richer data and easier insights are important for decision-making and might improve campaign effectiveness.
Ultimately, the integration of Marketing Cloud with Data Cloud relies on machine learning algorithms that refine themselves as more data comes in. This is an important feature, and the capacity for these systems to adapt to evolving conditions suggests an approach to marketing that can be more flexible and resilient in a changing world. I wonder about the specific aspects of data that trigger the adaptation and what factors are most influential in altering the machine learning model.
The development of this integration is a notable step in marketing technology. It has the potential to make data management more efficient and create more effective marketing campaigns. However, the successful implementation will require careful planning and attention to the potential ethical and practical implications of these new systems. It’s an intriguing area of development and one worth following closely to understand the impact of such integrated approaches to data and marketing.
Salesforce Marketing Cloud 7 Key Advancements in AI-Driven Personalization for 2025 - Introduction of AI-Based Engagement Scoring System
Salesforce Marketing Cloud's introduction of an AI-based engagement scoring system represents a step forward in understanding and optimizing customer interactions. This system uses machine learning to analyze a company's own customer data, particularly past behaviors, to predict how likely a customer is to engage with emails or mobile push messages. The system presents this information in an easy-to-understand format, enabling marketers to make better decisions about targeting and segmentation.
While this new system holds promise for improving the effectiveness of marketing efforts, it also raises some questions. How accurate are these AI-driven scores in predicting future engagement? And what are the implications for customer privacy as marketers increasingly rely on historical data to personalize messaging? It remains to be seen how businesses will navigate the trade-offs between leveraging these powerful tools and maintaining customer trust as these systems become more common. The challenge will be to use AI to enhance interactions without being overly intrusive or creating a sense of distrust.
Salesforce Marketing Cloud has introduced an AI-powered engagement scoring system that's designed to boost customer interactions. It uses a company's own customer data and machine learning to predict how likely someone is to interact with emails or mobile push messages. One of the more intriguing aspects is that it analyzes a wider range of data than previous systems, going beyond just simple metrics like email opens. Things like social media engagement, website activity, and even past interactions with customer service are all factored in, creating a more complete picture of how a customer interacts with a brand. This broader data view allows for more comprehensive engagement scoring, and initial research suggests it can be remarkably accurate – predicting customer behavior up to 80% of the time.
However, it's not just about prediction, it's also about agility. This system aims for real-time adjustments, meaning scores change based on actions in a matter of seconds. This allows marketers to tailor messages dynamically based on the latest customer interactions. One of the biggest benefits touted is a possible 30% improvement in campaign targeting. By understanding who's engaging with what, companies can potentially make campaigns much more relevant for different customer groups.
There's a layer of customization too – businesses can define which interactions are most important for scoring, allowing them to focus on the aspects of engagement that matter most to them. Furthermore, the use of machine learning means the system evolves as more data is collected, potentially getting smarter over time. It's like a self-improving feedback loop, where the AI continuously learns and adjusts its scoring criteria. This is promising, but also poses the question of how well it manages to avoid bias that might be present in the initial data it's trained on.
The ability to see shifts in engagement in real-time might allow companies to detect the early signs of customer disengagement. It could help identify potential churn and enable intervention strategies that potentially reduce the loss of customers by as much as 25%. The system even goes as far as trying to assess customer emotions. It’s a bit surprising to see this kind of emotional intelligence being baked into an engagement scoring system, and this aspect leads to the potential to change the tone and content of marketing communication dynamically, based on whether someone seems happy or unhappy.
Along with scoring, the AI aspect also creates more detailed reporting features. Marketers can get a better visualization of how engagement scores change over time, making it easier to see the effects of campaigns or understand how a customer's behavior is evolving. These are useful tools for strategy and potentially for assessing marketing ROI in real-time. It's exciting to see this advancement in engagement scoring, but as with any AI-driven system, it's important to consider the ethical considerations involved, particularly concerning data privacy and the potential for biased outcomes.
Salesforce Marketing Cloud 7 Key Advancements in AI-Driven Personalization for 2025 - Unified Customer Profile for Comprehensive Data Analysis
Salesforce Marketing Cloud's Unified Customer Profile represents a significant step forward in how companies understand their customers. It gathers a wide range of information about each individual, encompassing details like demographics, personality traits, and how they interact with a company across various touchpoints – both online and offline. Essentially, it creates a detailed picture of each customer, providing valuable insights that were previously fragmented. This comprehensive view becomes crucial for tailoring customer interactions in real-time. Marketers can leverage the profile to customize recommendations, promotional offers, and even a customer's overall journey based on their specific behavior and preferences across different marketing channels.
The integration of the Unified Customer Profile with Salesforce Data Cloud further strengthens the ability to analyze customer data. This integration makes advanced segmentation easier and empowers businesses to use AI-driven predictive analytics to better understand future customer needs and behaviors. This can lead to more targeted and effective marketing campaigns.
However, with the power to understand customers comes responsibility. It's important to recognize the potential ethical considerations and risks associated with this level of data collection and analysis. Questions about data privacy and the potential for bias in the algorithms used for prediction must be addressed. While the promise of highly personalized marketing is appealing, it's essential to implement these capabilities with a thoughtful approach that balances the needs of the business with the rights of the individual customer.
Salesforce Marketing Cloud's Unified Customer Profile offers a holistic view of each individual by combining all their interactions, both online and offline. It compiles a wide array of data, encompassing demographics, personality traits, and behaviors, essentially creating a snapshot of the typical customer within a specific target group. This profile is constructed using various data analysis techniques and incorporates engagement data collected across multiple channels, like website visits, app usage, preferences, and even where they came from in the first place.
The profile serves a critical purpose—it empowers real-time personalization and decision-making within marketing strategies. By knowing more about a specific customer, marketers can tailor promotional offers, recommendations, and the entire customer experience within tools like the Journey Builder. A major part of this is Salesforce's efforts to enhance customer engagement by using only the data the company already owns—what's referred to as "first-party data". It's a response to the rising awareness among marketers that customer data is central to creating meaningful experiences, with many acknowledging its significance in the development of effective marketing strategies.
Salesforce's Data Cloud has a major impact on this customer profile by uniting customer data from multiple sources. This broad integration allows for better and more sophisticated customer segmentation and creates the possibility for better, more targeted cross-channel marketing campaigns. AI and machine learning are embedded within the Data Cloud, leading to more advanced predictive analytics. This opens the door to a deeper understanding of customer needs and behaviors. And AI plays a vital role in this entire process, helping businesses make more data-driven decisions about their marketing strategies and, perhaps most importantly, humanize the way they communicate with customers.
In the pursuit of a holistic view of each customer, the Unified Customer Profile is a core component of what Salesforce calls "Customer 360". This approach utilizes advanced data integration methods to build a complete picture of customers, from their initial contact through all subsequent interactions.
While the advantages are readily apparent, it's not all smooth sailing. There are always potential drawbacks in working with sensitive customer information, and this approach necessitates careful consideration of privacy and the need to ensure the seamless integration of the new system with existing operations. It's a balancing act—providing personalized interactions while maintaining user trust and respecting privacy regulations. We need to look deeper into how well this technology handles individual customer differences and if there's any chance that biases might creep into the data used to build customer profiles. There’s also a need to investigate how it copes with changing regulations and evolving customer expectations.
Salesforce Marketing Cloud 7 Key Advancements in AI-Driven Personalization for 2025 - Adaptive Personalization Engines Tailored to Individual Customer Personas
Adaptive personalization engines are becoming increasingly vital for businesses seeking to understand and respond to the unique characteristics of their customers. These engines, powered by artificial intelligence, can dissect vast amounts of data to identify individual customer preferences and behaviors. This allows marketers to craft highly tailored messages and experiences that resonate with specific customer types or "personas."
By integrating diverse data sources, companies can develop a comprehensive understanding of each customer through features like the Unified Customer Profile, resulting in a more nuanced customer journey. This deeper comprehension leads to more precise targeting and content personalization across a range of marketing channels.
However, achieving truly adaptive personalization comes with its own set of challenges. Companies must carefully manage the potential for bias within AI algorithms and ensure they maintain a high level of transparency and respect for customer data privacy. As the pursuit of hyper-personalization intensifies, it's crucial for brands to find the balance between leveraging advanced AI tools for better marketing outcomes and upholding ethical considerations that safeguard the interests of the customer.
Adaptive personalization engines are becoming increasingly sophisticated in their ability to cater to individual customer needs. These engines are essentially AI-powered systems designed to understand and respond to the unique behaviors and preferences of each customer, going beyond simple demographics.
One of the intriguing aspects is how these systems can adapt in real-time. For example, if a customer repeatedly interacts with certain products on a website, the engine might instantly adjust their marketing interactions, delivering highly relevant recommendations in a matter of seconds. This real-time adaptation can create a more immediate and responsive marketing experience.
Beyond simple behavior, these engines are also becoming adept at generating and evolving customer "personas". By analyzing not just basic information like age and location, but also deeper behavioral patterns and even subtle emotional signals gleaned from engagement histories, a much more nuanced understanding of the customer emerges. This dynamic persona creation allows the engines to adjust their strategies as customers' preferences change over time, ensuring that marketing efforts always reflect the customer's current interests.
Furthermore, the ability to analyze data from a variety of sources is a key feature. These engines can draw insights from emails, social media, in-store interactions, and more. This multi-dimensional approach to data analysis provides a comprehensive picture of a customer, allowing for a more holistic understanding of their preferences and behaviors. This level of insight is crucial for developing highly targeted and relevant marketing strategies.
Another aspect of these engines that's worthy of attention is their predictive capabilities. By leveraging historical data such as past purchase behavior, these engines can make surprisingly accurate predictions about future customer behavior. For instance, they may be able to predict a customer's likelihood of responding to a specific promotion or engaging with certain content with up to 80% accuracy. This predictive accuracy can significantly improve the effectiveness of marketing campaigns.
Adding to the sophistication of these systems is their ability to consider contextual information. This means that the engine considers elements such as a customer's location or the device they are using when determining the most appropriate message to deliver. These contextual cues can significantly improve marketing relevance and timeliness, potentially leading to a more than 30% increase in customer engagement.
Beyond simple relevance, these engines are increasingly incorporating a form of "emotional intelligence" into their decision-making. By analyzing the tone and language of customer communications, these engines can start to gauge a customer's sentiment towards a brand, adapting the message accordingly. This subtle emotional awareness can improve customer experiences and enhance their feelings of being valued.
It's not just about interaction, it's about outcome. Many of these systems now have the capacity to simulate various customer engagement scenarios. By running hypothetical scenarios, businesses can explore the potential impact of their marketing campaigns before they launch them. This ability to see potential outcomes and refine strategies proactively might help significantly reduce wasted marketing spend—potentially by up to 20%.
Another facet of these systems that's proving useful is their capacity for risk mitigation. Some engines have sophisticated algorithms that can identify potential signs of customer dissatisfaction or impending churn. With the early warning capabilities, marketers can then intervene proactively to address concerns and hopefully decrease the likelihood of customers canceling services or leaving a brand. Research suggests these proactive interventions can lead to a reduction in customer churn of approximately 25%.
Given the increasing emphasis on customer privacy, advanced personalization engines are incorporating safeguards to ensure that compliance with regulations such as GDPR is not an afterthought. By carefully managing data and utilizing robust logging and protocol systems, these engines can help businesses more easily protect customer information and avoid potential privacy breaches.
Finally, the self-evolving nature of many personalization engines is a testament to the power of AI. These engines constantly refine their algorithms based on the data they collect. This means they become progressively better at predicting customer behavior over time and more adept at adapting to changes in market trends and consumer preferences. This constant learning and refinement ensures that marketing strategies remain relevant and effective in the long run.
While the benefits of adaptive personalization engines are enticing, it's essential to acknowledge the potential challenges and ethical concerns that arise with the use of these systems. Issues such as data privacy, the potential for algorithmic biases, and the need for human oversight in the development and implementation of these systems will require careful consideration as these technologies become more prevalent. This remains an area of active research and development as the balance between delivering highly personalized experiences and maintaining trust with customers is continuously being recalibrated.
Salesforce Marketing Cloud 7 Key Advancements in AI-Driven Personalization for 2025 - Automation Advancements for Streamlined Marketing Processes
**Automation Advancements for Streamlined Marketing Processes**
Salesforce Marketing Cloud is increasingly focused on automation to make marketing more efficient. This means automating tasks that were previously done manually, like creating and sending campaigns or personalizing customer journeys. The idea is to free up marketers to focus on more creative and strategic aspects of their jobs. While the potential for increased efficiency is huge, there's also a risk that marketing could become too automated, leading to a less personal and less human experience for customers. It's a balancing act—finding the right mix of automation to boost productivity without sacrificing the ability to connect with customers on a meaningful level. This need to combine efficient automation with human understanding and consideration is going to be a major challenge as these technologies develop.
Salesforce Marketing Cloud's advancements in AI are increasingly focused on using past customer interactions to better predict future behaviors. Research shows that using a customer's history can increase the accuracy of predicting engagement by up to 80%, which suggests a powerful role for continuous data in shaping marketing strategies.
These systems are also becoming increasingly dynamic in their ability to group customers. They can now adapt customer segments in real-time, based on how they're interacting with a business. This "dynamic segmentation" allows marketers to target individuals with more precision as their interests change, representing a more proactive approach to marketing compared to static segmentation.
It's not just email opens that are informing marketing actions now. AI-powered marketing systems are designed to draw insights from a variety of sources—from website visits to social media interactions to in-store activities. This "multimodal data" approach allows for a much richer understanding of each customer, leading to more tailored experiences and a greater ability to personalize engagement.
Some of the predictive analytics within these systems are surprisingly precise. Certain models can estimate customer behavior with remarkable accuracy, often within a 5% margin of error. This kind of precision can be a real asset in resource allocation, especially in identifying high-value customers and optimizing marketing spending.
A fascinating development is the incorporation of what might be called "emotional intelligence" into some automation systems. By analyzing customer sentiment from communications, they can now adapt their tone and messaging based on whether someone seems happy or frustrated. It's interesting to see how this capability can lead to more nuanced and thoughtful interactions, which may help to increase customer satisfaction.
Another interesting outcome is the speed at which these systems can react to customer actions. Many platforms can now automatically trigger marketing activities in real-time based on specific customer behaviors. This "behavioral trigger automation" can significantly reduce response times, potentially increasing engagement by over 30% as marketers deliver targeted messages immediately after key interactions.
We're also seeing an increase in the ability to model and simulate different marketing scenarios. Some platforms now have analytical tools that allow businesses to test various campaign ideas in a virtual environment before launching them, reducing the chances of failed campaigns by potentially 20%. It's like having a "what-if" tool for marketing strategies, leading to more informed decisions and better use of resources.
There's also a growing sophistication in identifying the signs of a customer who may be about to leave. Surprisingly effective churn analysis algorithms are able to identify the early warnings of customer disengagement. By spotting these signals, businesses can use retention strategies to potentially decrease churn by around 25%, which is a strong incentive to explore these tools.
The introduction of AI has also led to a revolution in how marketers can analyze campaign performance. The detailed visualizations that AI-driven reporting can provide give marketers insights into how engagement scores change over time. This allows for quicker analysis of marketing impact and easier monitoring of ROI. It's a useful tool for understanding how customer behavior evolves in response to marketing campaigns.
Interestingly, these newer marketing systems are increasingly designed to automatically handle data privacy regulations. This "compliance automation" not only safeguards customer information but also simplifies compliance with regulations like GDPR, enabling marketers to focus on strategy instead of legal hurdles.
This rapid evolution in AI-powered marketing automation brings significant potential, but also some concerns. It's crucial for marketers to consider the ethical and practical implications of these technologies to ensure they are implemented responsibly and in a way that respects customers. It's a developing area, but one with a potential to reshape the nature of marketing in the coming years.
More Posts from :