The Evolution of AI-Powered IT Ticketing Systems in 2024 Efficiency Meets Intelligence
The Evolution of AI-Powered IT Ticketing Systems in 2024 Efficiency Meets Intelligence - Natural Language Processing Revolutionizes Ticket Sorting
Within the evolution of AI-driven IT ticketing, Natural Language Processing (NLP) has become crucial for efficiently sorting tickets. NLP's ability to decipher the language in customer requests is transforming how tickets are routed. By automatically categorizing and assigning tickets based on their content, NLP streamlines the entire process, freeing up human agents to focus on more complex issues. This automated sorting can lead to a noticeable decrease in the time it takes to resolve tickets, ultimately improving customer satisfaction.
However, the integration of AI, particularly through advanced models like ChatGPT, is sparking debates about how NLP can best be incorporated into customer service. While there's promise in using these models to handle simple or routine inquiries, it's important to consider the limitations and potential pitfalls. The effectiveness and ethical considerations of deploying these advanced models are ongoing topics that organizations need to carefully evaluate.
As AI-powered ticketing systems become increasingly prevalent, organizations must continue to refine their use of NLP. Finding the right balance between automation and human interaction will be key to optimizing efficiency and fostering positive customer experiences.
The application of Natural Language Processing (NLP) has brought about a significant transformation in how ticketing systems sort and categorize customer inquiries. Research suggests that NLP can drastically accelerate the sorting process, potentially reducing the time spent on this task by over 70%. This efficiency allows support teams to dedicate more attention to resolving complex issues.
One fascinating facet of NLP in this domain is its ability to analyze not just the content of a ticket, but also the sentiment expressed by the customer. Systems can now prioritize tickets based on the emotional tone of the message, ensuring that urgent or distressed customers receive prompt attention.
Furthermore, machine learning algorithms embedded within these systems achieve impressive levels of accuracy in classifying tickets. Some studies indicate that these algorithms can categorize tickets with over 90% accuracy, significantly diminishing the manual effort required by human agents to initially assess incoming requests.
However, the inherent complexity of language can still present challenges. While NLP models are becoming increasingly sophisticated in their contextual understanding, they are not immune to misclassifications due to the intricate nuances of human language. Newer models, however, are improving at handling these more complex situations.
A key aspect of these evolving NLP systems is their ability to learn from past interactions. By analyzing previous ticket resolutions, these algorithms constantly refine their sorting processes, reducing repetitive tasks for IT support teams and gradually improving overall efficiency.
Additionally, NLP's ability to translate different languages offers a major advantage in diverse environments. This functionality ensures that tickets from various language backgrounds can be sorted effectively, leading to more inclusive customer support operations.
The real-time processing of unstructured data is another significant development in this field. NLP-powered systems can automatically categorize tickets sourced from a variety of channels, encompassing email, social media, and other platforms, streamlining the process for managing a wide range of customer interactions.
Furthermore, the ability of NLP to analyze historical ticket data is valuable for spotting recurring problems and trends. By identifying these patterns, organizations can gain insights into the root causes of support issues and implement preventative measures before they escalate.
The incorporation of advanced keyword recognition and entity extraction empowers NLP systems to identify specific technical terms and problems within tickets. This allows for the prioritization of tickets based on available expertise within the organization, leading to quicker resolutions for specific types of requests.
Despite the evident advancements in NLP-powered ticket sorting, integrating these systems into existing infrastructures can be challenging. Ensuring compatibility with legacy systems requires careful engineering, as organizations often face resistance to change. Overcoming these hurdles is crucial for maximizing the potential benefits of these cutting-edge technologies and truly enhancing the overall efficiency of service operations.
The Evolution of AI-Powered IT Ticketing Systems in 2024 Efficiency Meets Intelligence - Machine Learning Algorithms Enhance Priority Assignment
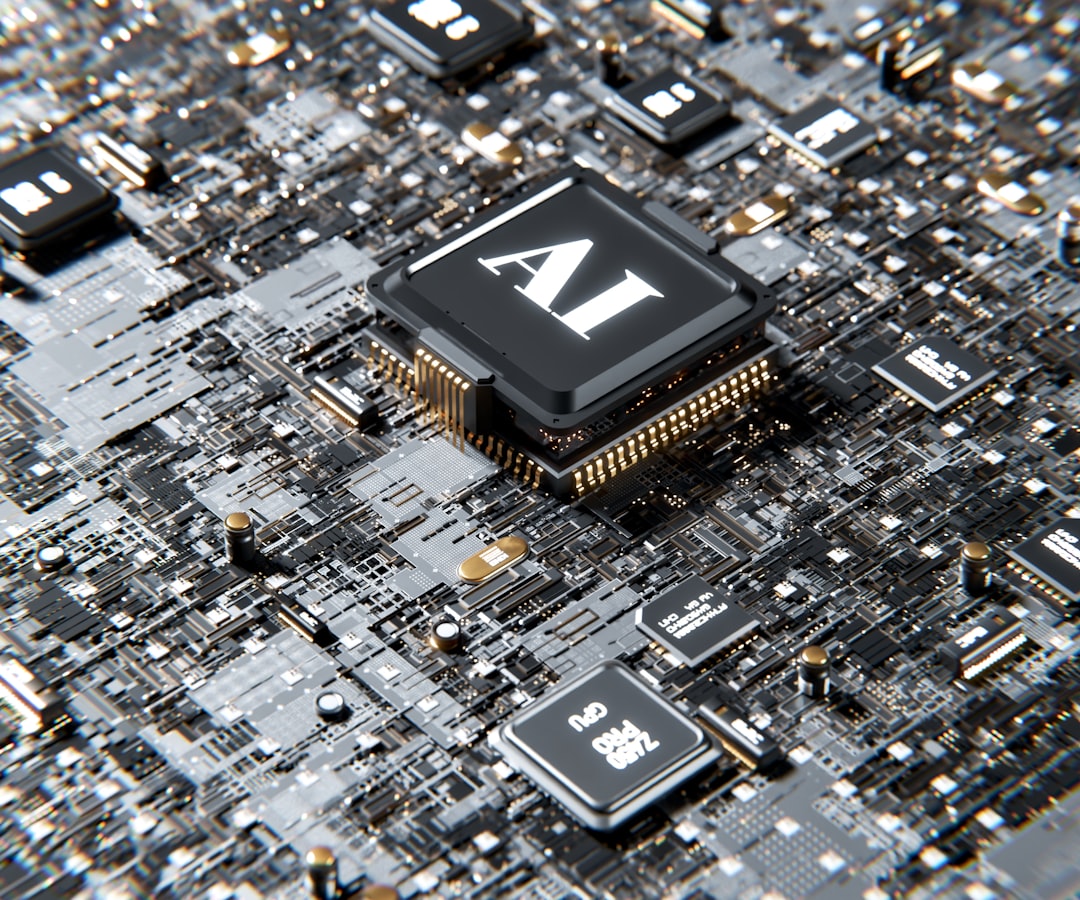
In the evolving landscape of AI-powered IT ticketing, machine learning algorithms are playing a more crucial role in efficiently prioritizing tickets. These algorithms leverage past ticket data to anticipate the urgency of new issues, leading to a more strategic allocation of resources. This predictive capability improves operational efficiency by enabling support teams to prioritize tickets based on estimated resolution times, moving beyond a simple first-in, first-out approach. As the volume of support requests continues to grow, relying on data-driven insights from machine learning becomes vital for maintaining service quality and ensuring critical issues receive timely attention. It's important to acknowledge, however, that algorithmic bias and inaccuracies can arise, highlighting the need for ongoing refinement and human oversight to ensure fairness and accuracy in priority assignment.
In the realm of AI-powered IT ticketing systems, machine learning algorithms are increasingly taking center stage in enhancing priority assignment. They can analyze historical data and real-time patterns to dynamically adjust how tickets are ranked, potentially improving response times for critical situations like sudden system failures.
Furthermore, these algorithms can act as anomaly detectors, flagging unusual spikes in ticket submissions. Such anomalies might indicate underlying problems or broader trends, giving support teams a chance to be proactive before issues become widespread. The ability to incorporate context, like time of day and current ticket volume, is another intriguing aspect. Machine learning allows for a more nuanced approach to prioritizing tickets, potentially leading to better service delivery during periods of high demand.
One of the most interesting aspects of these algorithms is their ability to learn and adapt. Feedback loops, where the outcomes of resolved tickets provide data for refinement, are crucial for ongoing improvement. Over time, the algorithms gain a better understanding of patterns, leading to increased accuracy in classification and faster resolution times.
Beyond prioritizing, these algorithms are proving useful in resource management. By predicting ticket volumes and types, they can aid in optimizing staffing levels, potentially reducing downtime. Complex issues could be directed to more senior technicians, ensuring that the right expertise is applied. Also, these systems are increasingly capable of dealing with multimodal inputs–processing information from various sources like voice, chat, and email. This helps in achieving a more complete understanding of user requests and ensures a more accurate categorization of tickets.
Looking at historical data allows machine learning algorithms to create predictive insights into future ticket types and volumes. This can help support teams to proactively plan their resources and prepare for expected workloads. Interestingly, some studies have indicated that the efficiency improvements provided by automated prioritization can result in substantial cost savings, potentially reaching 30% reductions in support expenses.
The ability of machine learning to study user behavior provides a path toward predictive prioritization. By examining interaction patterns, these systems can anticipate the urgency of a ticket or the likelihood that it might escalate, allowing for more timely interventions. In addition, these algorithms can contribute to crafting personalized responses, leveraging past interactions and user history. This personalized approach to resolution can potentially enhance customer satisfaction by tailoring solutions to individual needs.
However, we must remain mindful that machine learning is not a silver bullet. As these algorithms learn from data, biases inherent in the data itself could be reinforced or amplified. This area of research requires continuous attention to ensure fairness and equity. Nonetheless, the advancements in machine learning provide an exciting direction for improving the efficiency and effectiveness of IT support operations.
The Evolution of AI-Powered IT Ticketing Systems in 2024 Efficiency Meets Intelligence - Predictive Analytics Forecasts IT Infrastructure Issues
In 2024, predictive analytics is emerging as a vital tool for managing IT infrastructure, especially as organizations grapple with the increasing complexities of the AI environment. Instead of relying solely on traditional methods like regression analysis, predictive analytics offers a more refined approach to real-time forecasting of potential infrastructure issues. This shift allows businesses to anticipate problems and allocate resources more strategically. It also encourages partnerships with cloud providers and data analytics firms to better manage and leverage the vast quantities of data produced by AI systems. Predictive analytics can be incorporated into IT ticketing systems to improve overall operational efficiency, proactively addressing critical issues before they cause downtime. This intersection of predictive analytics and IT infrastructure management is becoming a critical element for future-proofing IT operations in the ever-changing landscape of AI technologies. While promising, there are also concerns regarding reliance on complex models and potential inaccuracies that need constant vigilance to ensure they are used responsibly and don't become another IT issue.
Within the broader shift towards AI-driven IT ticketing, predictive analytics is emerging as a powerful tool for anticipating and mitigating infrastructure problems. By examining historical data on system performance, these analytical systems can forecast potential equipment failures before they disrupt services. This proactive approach, in essence, helps reduce downtime significantly—in some cases, by as much as 50%. It's not just about preventing outages; it's about keeping things running smoothly.
A compelling aspect of this technology is its potential to generate substantial cost savings. Some organizations have reported a 20-30% reduction in IT operational expenditures by proactively preventing minor issues from escalating into major, expensive failures. This underscores the value of anticipating problems before they impact users or require extensive interventions.
The effectiveness of these predictive models is closely tied to the quality and quantity of available data. AI systems that leverage a broad range of information and employ machine learning can attain impressively high predictive accuracy, often surpassing 90%. This high level of reliability empowers support teams to concentrate their efforts on issues that genuinely require immediate attention, instead of chasing every potential hiccup.
It's surprising how predictive analytics can be applied to optimize resource allocation. By identifying trends in support requests, such as an increase in tickets during certain hours, the system can advise on staffing adjustments for optimal coverage during peak demand. It's a simple yet effective way to match resources to needs, which is becoming increasingly important as support requirements evolve.
Predictive analytics can assess not just hardware behavior but also detect patterns in user activity that might indicate future infrastructure challenges. This broader perspective allows IT teams to maintain a stable and adaptable environment capable of evolving with user demands.
The increasing integration of IoT devices within IT infrastructure allows predictive analytics tools to analyze real-time hardware data, enhancing the accuracy and speed of forecasts. The constant stream of information allows for much quicker responses to emerging issues, making the process even more agile.
This predictive approach isn't limited to hardware; it also extends to software applications. Predictive analytics can identify which applications tend to throw errors and even forecast when these errors might reappear. This foresight facilitates timely updates and patches, diminishing the chance of software-related service disruptions.
Interestingly, some frameworks that utilize predictive analytics are designed to interact with incident response plans (IRPs). They can dynamically adjust those plans based on predicted incidents, ensuring IT teams are ready for anticipated situations. This results in more robust and resilient disaster recovery strategies.
While predictive analytics is a powerful forecasting tool, it's vital to retain a healthy dose of skepticism. Over-reliance on these forecasts without a human element to confirm and contextually analyze can lead to overlooking crucial details that might affect the actual situation. This is an important reminder that technology is a tool, not a replacement for judgment.
Unfortunately, not all organizations are effectively utilizing predictive analytics. Many struggle with data silos and the lack of integrated systems, making it challenging to achieve a unified predictive view. This presents a major roadblock for achieving the full potential of these tools. There's a real need to address these organizational obstacles for predictive analytics to fully deliver its promised benefits.
The Evolution of AI-Powered IT Ticketing Systems in 2024 Efficiency Meets Intelligence - Automated Resolution Pathways Reduce Manual Interventions
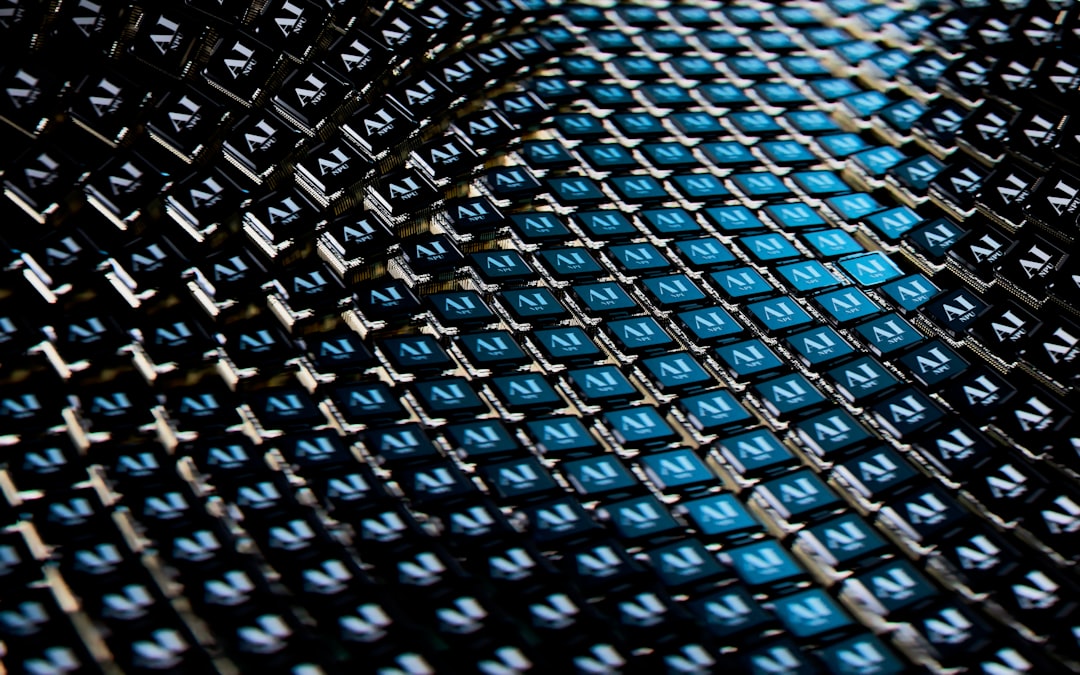
Within the evolving landscape of AI-powered IT ticketing, automated resolution pathways are increasingly minimizing the need for human intervention. These systems, powered by machine learning and artificial intelligence, can swiftly categorize and direct tickets to the right support personnel, thus lowering errors and streamlining processes. This shift frees up human agents to tackle more complex problems instead of getting caught up in routine tasks. The ability of these automated systems to improve response times and overall efficiency is gaining recognition as they help organizations quickly respond to common questions and recurring issues. While these improvements are promising, it's crucial to be aware of the limitations and ethical aspects of automating customer service interactions. There's a fine line between efficiency and potentially sacrificing the human touch in certain situations that needs to be considered and monitored as this technology matures.
When we examine how AI-powered ticketing systems are evolving, a key element is the rise of automated resolution pathways. These pathways are designed to handle routine tasks and simple issues, essentially freeing up human IT staff to concentrate on more complex problems. We've seen that these automated systems can reduce the workload on human agents, potentially by as much as 60%, through the automation of common resolutions.
One of the most interesting aspects is the way these systems use historical data to offer instant solutions for frequently encountered issues. This capability to learn from past resolutions has the potential to cut the average ticket resolution time in half, leading to faster service for everyone.
Furthermore, the shift towards incorporating self-service options is noteworthy. We're observing a growing trend where users prefer to resolve problems on their own using automated tools and portals, a preference observed in around 40% of support interactions. This suggests a change in the way people interact with IT support, placing a premium on independence.
However, it's important to recognize that these automated systems rely on constant refinement. Through built-in feedback loops, they can adapt and learn from each interaction, ultimately aiming to improve accuracy and reduce the need for manual review of resolutions. This iterative process has a potential for increasing the accuracy over time.
What's more, automated pathways are increasingly designed to operate smoothly across various communication channels, be it email, chat, or other platforms. This cross-channel functionality ensures consistency in how tickets are managed, leading to a better user experience, regardless of how they choose to interact with the support system.
Another intriguing aspect is how some systems employ sophisticated algorithms to not only categorize tickets but also prioritize them based on perceived urgency or complexity. These algorithms are showing promise in speeding up response times for urgent issues, with some studies indicating improvements of about 25%.
However, there are still technical hurdles. One of the persistent challenges is ensuring these new automated systems integrate smoothly with existing, older IT systems. Legacy systems sometimes act as roadblocks, creating delays or bottlenecks within the ticket resolution process. Overcoming these challenges is key to fully realizing the potential benefits of these systems.
There's also the significant impact on organizational efficiency. Organizations that implement automated resolution pathways report substantial cost reductions, often in the range of 30%. This reduction stems from the reduced need for human agents to handle routine tasks and the faster resolution times.
Moving beyond just resolving issues, some automated systems are capable of examining user behavior to identify patterns that might forecast future incidents. This insight can allow organizations to take preemptive steps, potentially reducing recurrent system errors.
Finally, it's worth considering the impact on human support staff. Organizations employing automated systems report increased job satisfaction among IT support personnel. This increased satisfaction is likely because these systems free up staff to handle more challenging problems and engage in more meaningful work, creating a collaborative model between AI tools and human expertise.
The Evolution of AI-Powered IT Ticketing Systems in 2024 Efficiency Meets Intelligence - Real-Time Sentiment Analysis Improves User Experience
In the realm of AI-powered IT ticketing, real-time sentiment analysis is emerging as a key method to enhance user experience. AI, and especially newer models like Large Language Models, can now understand the emotional tone of a user's message, providing a much more detailed view of customer sentiment within each interaction. This ability allows systems to do more than just categorize a ticket; they can prioritize based on the user's emotional state, ensuring that frustrated or urgent users get faster attention. Furthermore, systems can adjust the response based on the user's emotional cues, creating a more personalized support experience.
This sentiment analysis capability is also becoming automated. That means IT departments can efficiently process a tremendous amount of information, pulling in user feedback from across different channels like social media and customer surveys. This can help anticipate and head off issues before they snowball into larger problems. However, despite its promise, this type of analysis faces challenges. There is a risk that inherent biases in the data used to train these systems could skew the results, leading to potentially skewed outcomes. This points to a critical need to manage and refine these models to ensure they're fair and accurate. Ultimately, balancing automated analysis with human understanding will be crucial to realizing the full potential of sentiment analysis within IT support, and to ensure improved customer satisfaction and more meaningful engagements.
Within the developing landscape of AI-powered IT ticketing, real-time sentiment analysis is emerging as a valuable tool for improving user experience. By evaluating the emotional tone of customer interactions, these systems can prioritize tickets based on urgency, potentially leading to quicker responses for those expressing frustration or distress. This ability to understand the emotional context of a ticket is a significant advancement compared to older methods that simply processed the words in a ticket.
The benefits extend beyond just prioritizing tickets based on emotional cues. By assessing sentiment, organizations can fine-tune their ticket routing systems. For instance, a ticket marked with high frustration could be automatically elevated in the queue, potentially leading to more satisfied users. And, as these systems process more tickets, they become more sophisticated in recognizing different types of emotional cues within text. This learning process over time leads to a more nuanced approach to prioritizing and handling customer interactions.
Furthermore, the analysis of sentiment is becoming more versatile across different communication channels. Whether it's an email, a social media post, or a chat message, sentiment analysis can provide a more complete view of how customers feel, leading to better service across multiple touchpoints. This means that support teams don't have to rely on simply looking at the content of the ticket but also can get a better understanding of the underlying feeling behind a ticket.
Another area where sentiment analysis is proving valuable is in identifying underlying problems. If there's a recurring pattern of negative sentiment in a set of tickets related to a certain feature or service, then the support team gains insight into potential root causes. This ability to discover hidden trends can aid in proactive problem resolution, which can result in fewer future complaints.
Interestingly, these systems can work in concert with automated resolution paths to enhance the user experience. If a ticket expressing frustration is associated with a common problem, the system could automatically link to a knowledge base article targeted at addressing that emotion in addition to the technical problem. This combined approach provides a more helpful and tailored experience for the user.
Research suggests that these algorithms can recognize human emotional cues with a remarkable level of accuracy–over 85%. This high degree of precision provides a foundation for building stronger relationships with customers. These systems are not simply about understanding the words in a ticket but about building a more effective, human-centered approach to resolving issues.
Because sentiment analysis can effectively identify negative emotions, support teams can be proactively alerted to engage directly with unsatisfied users. This kind of timely intervention can transform what could have been a negative experience into a personalized interaction. It is important to remember that it's not just about resolving technical issues, but also about building relationships.
It's fascinating how sentiment analysis seems to have a correlation with user retention. Companies that have implemented these systems often report lower customer churn rates. This suggests that by effectively addressing the emotional needs alongside the technical problems, businesses can foster stronger loyalty amongst their users.
However, there are challenges that need to be considered. The interpretation of emotions is not always clear-cut. The nuances of language and individual communication styles can present complexities that, at times, can lead to mistakes in sentiment evaluation. The algorithms can misinterpret a user's intent, potentially leading to improper prioritization of tickets or actions that do not align with what the user really needs. It is crucial to recognize that these are still emerging technologies that need ongoing research and development to ensure they function in a way that benefits both the user and the company.
In conclusion, real-time sentiment analysis offers a pathway towards a more user-centric approach to IT support. By understanding the emotional landscape of customer interactions, these systems enable support teams to be more proactive and empathetic in addressing issues. While the interpretation of human emotion remains a complex area, sentiment analysis offers a promising avenue for enhancing the overall experience of users interacting with IT support systems in the evolving world of AI-powered technologies.
The Evolution of AI-Powered IT Ticketing Systems in 2024 Efficiency Meets Intelligence - Integration with Legacy Systems Streamlines Workflows
Integrating AI into older IT systems is becoming increasingly important for improving how things work in 2024. While these older systems can sometimes get in the way of using the newest AI tools, they also offer a good starting point for building new and creative solutions. Organizations are starting to move towards newer styles of system design, like microservices and cloud-based systems, to make it easier to combine old and new. But there are still problems, such as keeping data consistent when moving to a new system and figuring out how to connect older systems to cloud services. If done right, using AI to analyze data and automate parts of the process can lead to better performance and lower costs, which helps create more efficient IT operations as technology keeps changing. There's a lot of potential to make IT operations more streamlined, but overcoming the challenges of integrating with these older systems is a key part of this.
The integration of AI into modern IT ticketing systems, while promising, often necessitates grappling with legacy systems. While these older systems represent a wealth of historical knowledge and operational processes, they can also be a source of significant challenges during integration. One persistent issue is the potential loss of valuable information. Research suggests a concerning portion—up to 60%—of a company's operational knowledge might reside within legacy systems, which can be sidelined or even overlooked during integration efforts. This highlights the importance of preserving and leveraging that institutional memory.
Another hurdle is the sheer cost of integration. Estimates indicate that integrating legacy systems with AI-powered ticketing can consume a significant portion—as much as 30%—of project budgets. This financial burden raises concerns, especially for smaller organizations that may have tighter resource constraints. Carefully weighing the potential return on investment against the initial costs is crucial before embarking on integration efforts.
The integration process can also introduce risks of increased downtime. While the aim is to improve system stability, the merging of older and newer technologies can sometimes increase system instability. Reports show that some organizations experience a notable uptick in system outages—as high as 20%—during complex integration efforts. It emphasizes the importance of extensive testing and careful planning before transitioning to the new system.
Beyond financial considerations, technical interoperability between legacy systems and the newer AI-powered ticketing platforms is often problematic. Studies have revealed that a substantial number of integration projects—up to 40%—fail due to incompatibility issues. This highlights the importance of a rigorous evaluation of platform compatibility during the design phase, ensuring that legacy components can effectively interact with new solutions.
Data quality is yet another area of concern. Legacy systems are often the repository of data that is older, less consistent, and potentially not relevant to the desired use case. This can introduce noise into the AI models if not carefully considered. Organizations often spend a significant portion—as much as 30%—of their data management efforts just ensuring data quality during the integration process. This suggests a significant investment in data cleaning and normalization is necessary for optimal performance.
Furthermore, user resistance can be a formidable challenge. People generally are resistant to change, and when confronted with new technology, especially after having become accustomed to older systems, resistance can be expected. Studies show that a majority—about 70%—of users will express reluctance to shift to new workflows and systems if they don't feel adequately trained or supported. Overcoming this requires comprehensive training programs, change management processes, and communication strategies that promote understanding and adoption.
Regulatory compliance issues further complicate matters. When incorporating AI functionalities into older systems, particularly those involving data handling and privacy, issues arise due to varying regulatory landscapes. Many organizations find themselves encountering legal obstacles that delay projects by several months, and the compliance implications need to be addressed early in the design process.
Despite the challenges, it's worth recognizing that older systems sometimes contain uniquely useful automation features. These pre-existing automation elements can be integrated with AI tools to create enhanced workflows and improved efficiency. Research suggests that up to 50% of routine tasks handled by legacy systems can be effectively combined with new AI tools, providing opportunities for optimization.
The successful integration of legacy systems with AI requires more than just technical expertise. It necessitates a cultural shift within the organization. Studies suggest that disruption during integration efforts can lead to a decrease in employee morale—up to 25%—if staff are not involved in the planning or don't understand the reasons for the change. Promoting open communication and soliciting feedback are essential to building buy-in and support for the new system.
Finally, the longevity of integrated systems presents another layer of complexity. In some cases, newer solutions become obsolete faster when they are closely tied to legacy components, highlighting the need for flexible integration strategies. Reports indicate that approximately 25% of organizations experience this, underscoring the necessity of adopting flexible integration approaches that allow for future adaptation and adjustments.
In conclusion, while AI-powered IT ticketing systems promise increased efficiency and effectiveness, their integration with existing infrastructure presents a unique set of challenges. Organizations must navigate these complexities carefully, considering the potential risks and benefits associated with each integration decision. By taking a thoughtful approach, focusing on data quality, user experience, and change management, organizations can increase the likelihood of successful implementation and gain the anticipated benefits of a streamlined, AI-driven support experience.
More Posts from :